Anthropic's Claude recently gained attention with new capabilities allowing it to access and synthesize real-time web information, effectively acting as a research assistant. This feature, often discussed as "Claude Research," aims to go beyond simple web search by exploring multiple angles of a topic, pulling together information from various sources, and delivering synthesized answers. While powerful, relying on closed-source, proprietary systems isn't always ideal. Many users seek more control, transparency, customization, or simply want to experiment with the underlying technology.
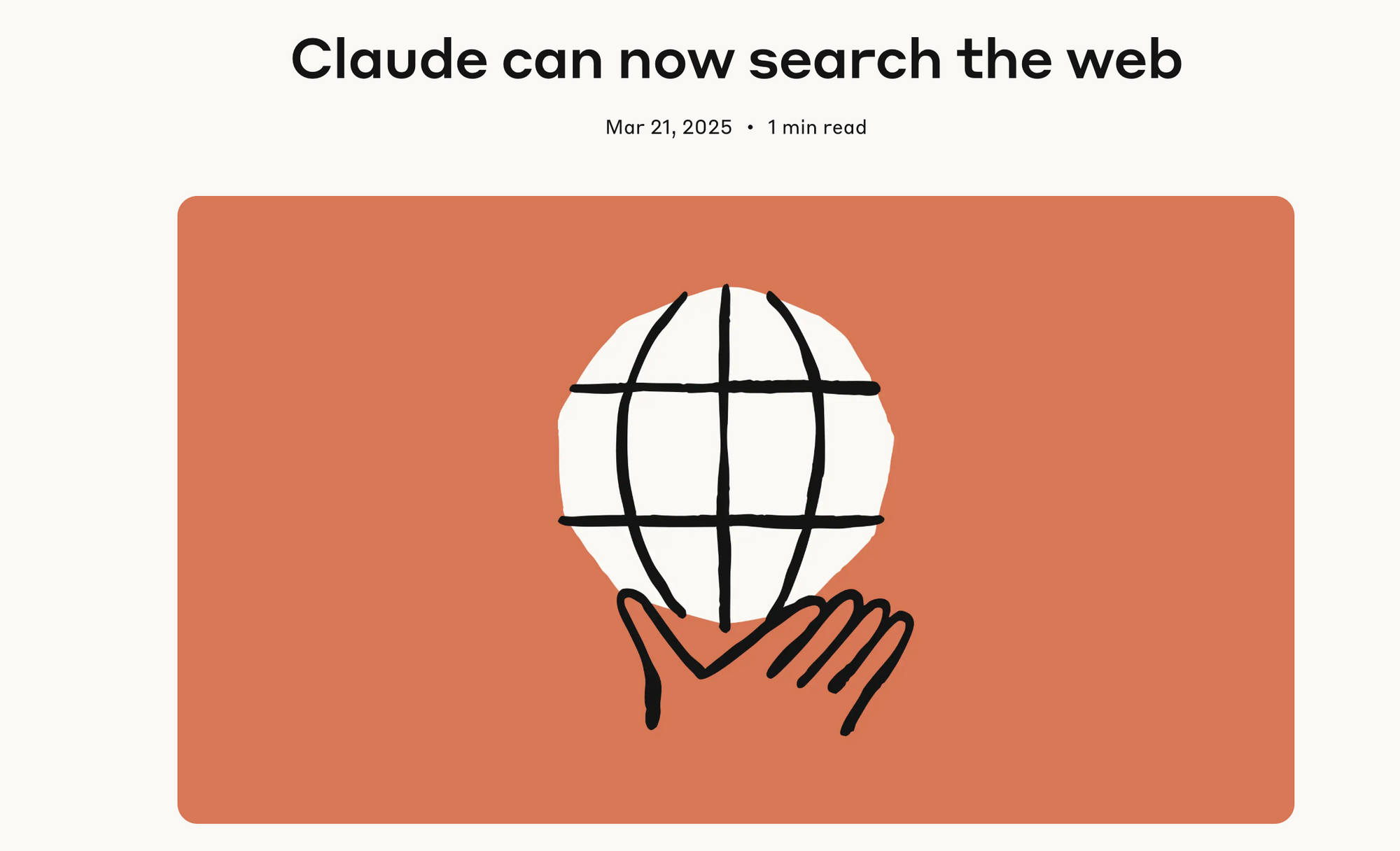
The good news is that the open-source community often provides building blocks to replicate such functionalities. One interesting project in this space is btahir/open-deep-research
on GitHub. This tool aims to automate the process of conducting in-depth research on a topic by leveraging web searches and Large Language Models (LLMs).
Let's first understand the key capabilities offered by sophisticated AI research features like Claude's, which open-deep-research
attempts to emulate in an open-source fashion, and then dive into how you can run this tool yourself.
Introducing open-deep-research
: Your Open-Source Starting Point
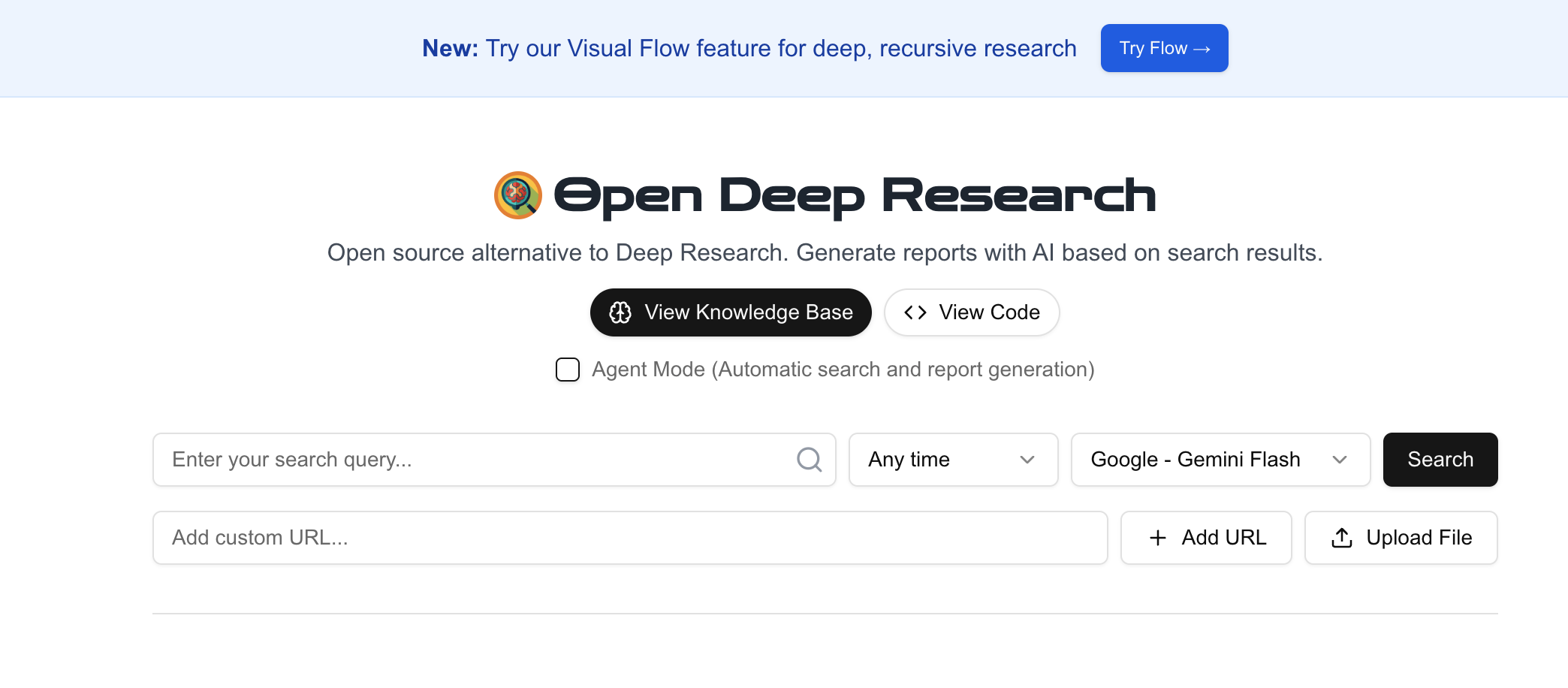
The open-deep-research
project (https://github.com/btahir/open-deep-research) provides a framework to achieve similar goals using readily available tools and APIs. It likely orchestrates a pipeline involving:
- Search Engine Queries: Using APIs (like SearchApi, Google Search API, etc.) to find relevant web pages for a given research topic.
- Web Scraping: Fetching the content from the identified URLs.
- LLM Processing: Utilizing a Large Language Model (commonly via the OpenAI API, but potentially adaptable) to read, understand, synthesize, and structure the information gathered from the web pages.
- Report Generation: Compiling the processed information into a final output, such as a detailed report.
By running this yourself, you gain transparency into the process and the ability to potentially customize it.
Want an integrated, All-in-One platform for your Developer Team to work together with maximum productivity?
Apidog delivers all your demans, and replaces Postman at a much more affordable price!
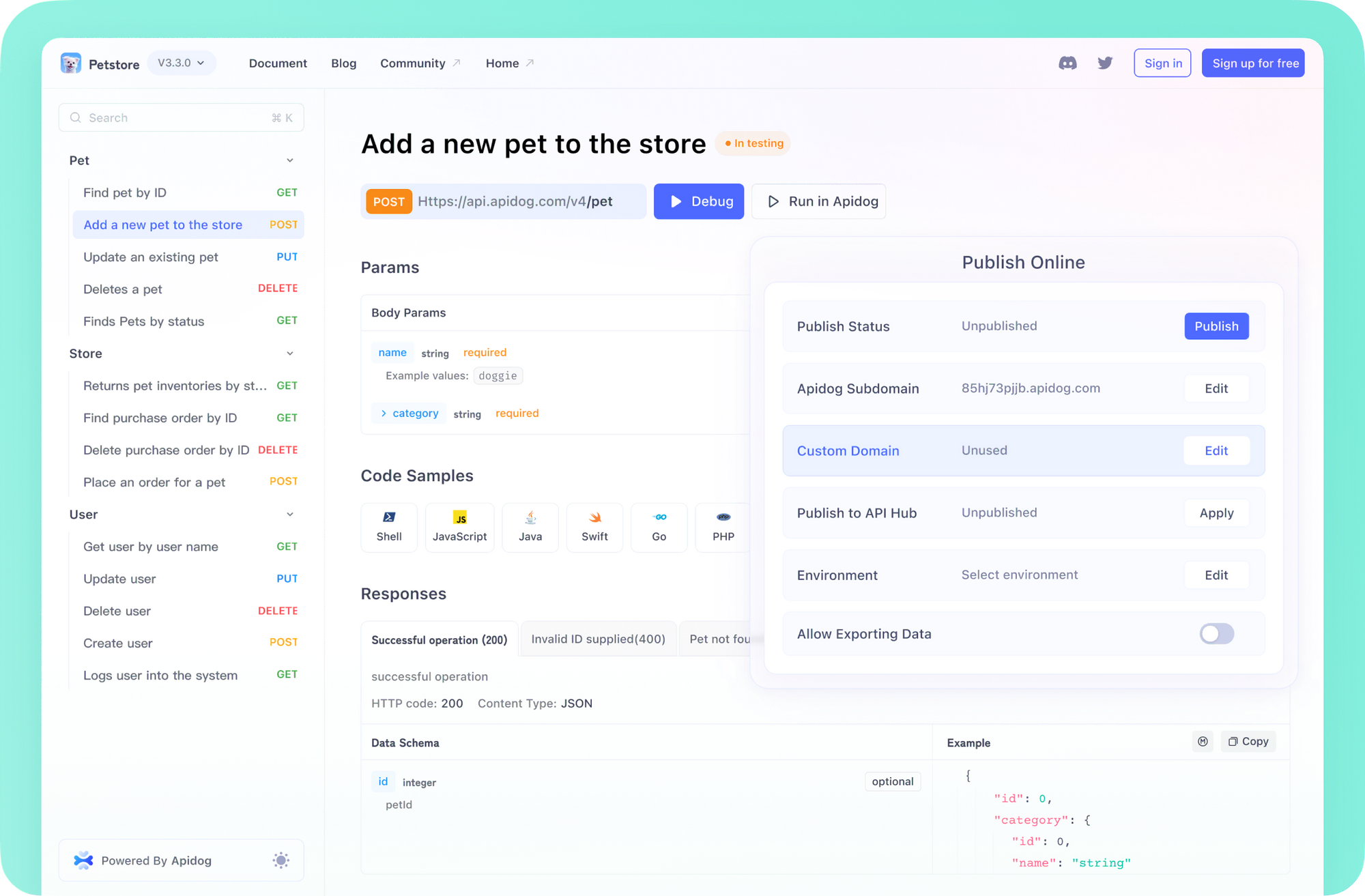
Step-by-Step Guide to Running open-deep-research
Ready to try building your own research assistant? Here’s a detailed guide to getting open-deep-research
up and running.
Prerequisites:
- Python: You'll need Python installed on your system (usually Python 3.7+).
- Git: Required for cloning the repository.
- API Keys: This is crucial. The tool will need API keys for:
- A Search Engine API: To perform web searches programmatically. Examples include SearchApi, Serper, or potentially others depending on the project's configuration. You'll need to sign up for one of these services and get an API key.
- An LLM API: Most likely, the OpenAI API key for accessing GPT models (like GPT-3.5 or GPT-4) will be required for the synthesis step. You'll need an OpenAI account with API access.
- (Check the
open-deep-research
README for the specific required APIs and keys). - Command Line / Terminal: You'll be running commands in your terminal or command prompt.
Step 1: Clone the Repository
First, open your terminal and navigate to the directory where you want to store the project. Then, clone the GitHub repository:
git clone <https://github.com/btahir/open-deep-research.git>
Now, change into the newly created project directory:
cd open-deep-research
Step 2: Set Up a Virtual Environment (Recommended)
It's best practice to use a virtual environment to manage project dependencies separately.
On macOS/Linux:
python3 -m venv venv
source venv/bin/activate
On Windows:
python -m venv venv
.\\venv\\Scripts\\activate
Your terminal prompt should now indicate that you are in the (venv)
environment.
Step 3: Install Dependencies
The project should include a requirements.txt
file listing all the necessary Python libraries. Install them using pip:
pip install -r requirements.txt
This command will download and install libraries such as openai
, requests
, potentially beautifulsoup4
or similar for scraping, and libraries for the specific search API used.
Step 4: Configure API Keys
This is the most critical configuration step. You need to provide the API keys you obtained in the prerequisites. Open-source projects typically handle keys via environment variables or a .env
file. Consult the open-deep-research
README file carefully for the exact environment variable names required.
Commonly, you might need to set variables like:
OPENAI_API_KEY
SEARCHAPI_API_KEY
(orSERPER_API_KEY
,GOOGLE_API_KEY
etc., depending on the search service used)
You can set environment variables directly in your terminal (these are temporary for the current session):
On macOS/Linux:
export OPENAI_API_KEY='your_openai_api_key_here'
export SEARCHAPI_API_KEY='your_search_api_key_here'
On Windows (Command Prompt):
set OPENAI_API_KEY=your_openai_api_key_here
set SEARCHAPI_API_KEY=your_search_api_key_here
On Windows (PowerShell):
$env:OPENAI_API_KEY="your_openai_api_key_here"$env:SEARCHAPI_API_KEY="your_search_api_key_here"
Alternatively, the project might support a .env
file. If so, create a file named .env
in the project's root directory and add the keys like this:
OPENAI_API_KEY=your_openai_api_key_here
SEARCHAPI_API_KEY=your_search_api_key_here
Libraries like python-dotenv
(if listed in requirements.txt
) will automatically load these variables when the script runs. Again, check the project's documentation for the correct method and variable names.
Step 5: Run the Research Tool
With the environment set up, dependencies installed, and API keys configured, you can now run the main script. The exact command will depend on how the project is structured. Look for a primary Python script (e.g., main.py
, research.py
, or similar).
The command might look something like this ( check the README for the exact command and arguments!):
python main.py --query "Impact of renewable energy adoption on global C02 emissions trends"
Or perhaps:
python research_agent.py "Latest advancements in solid-state battery technology for electric vehicles"
The script will then:
- Take your query.
- Use the search API key to find relevant URLs.
- Scrape content from those URLs.
- Use the OpenAI API key to process and synthesize the content.
- Generate an output.
Step 6: Review the Output
The tool will likely take some time to run, depending on the complexity of the query, the number of sources analyzed, and the speed of the APIs. Once finished, check the output. This might be:
- Printed directly to your terminal console.
- Saved as a text file or Markdown file in the project directory (e.g.,
research_report.txt
orreport.md
).
Review the generated report for relevance, coherence, and accuracy.
Customization and Considerations
- LLM Choice: While likely defaulting to OpenAI, check if the project allows configuring different LLMs (perhaps open-source models running locally via Ollama or LM Studio, though this would require code changes if not built-in).
- Search Provider: You might be able to swap out the search API provider if needed.
- Prompt Engineering: You could potentially modify the prompts used to instruct the LLM during the synthesis phase to tailor the output style or focus.
- Cost: Remember that using APIs (especially OpenAI's more powerful models and potentially search APIs) incurs costs based on usage. Monitor your spending.
- Reliability: Open-source tools like this might be less robust than commercial products. Websites change, scraping can fail, and LLM outputs can vary. Expect to potentially debug issues.
- Complexity: Setting this up requires more technical effort than using a polished SaaS product like Claude.
Conclusion
While commercial AI tools like Claude offer impressive, integrated research capabilities, open-source projects like btahir/open-deep-research
demonstrate that similar functionalities can be built and run independently. By following the steps above, you can set up your own automated research agent, giving you a powerful tool for deep dives into various topics, combined with the transparency and potential for customization that open source provides. Remember to always consult the specific project's documentation (README.md
) for the most accurate and up-to-date instructions. Happy researching!
Want an integrated, All-in-One platform for your Developer Team to work together with maximum productivity?
Apidog delivers all your demans, and replaces Postman at a much more affordable price!
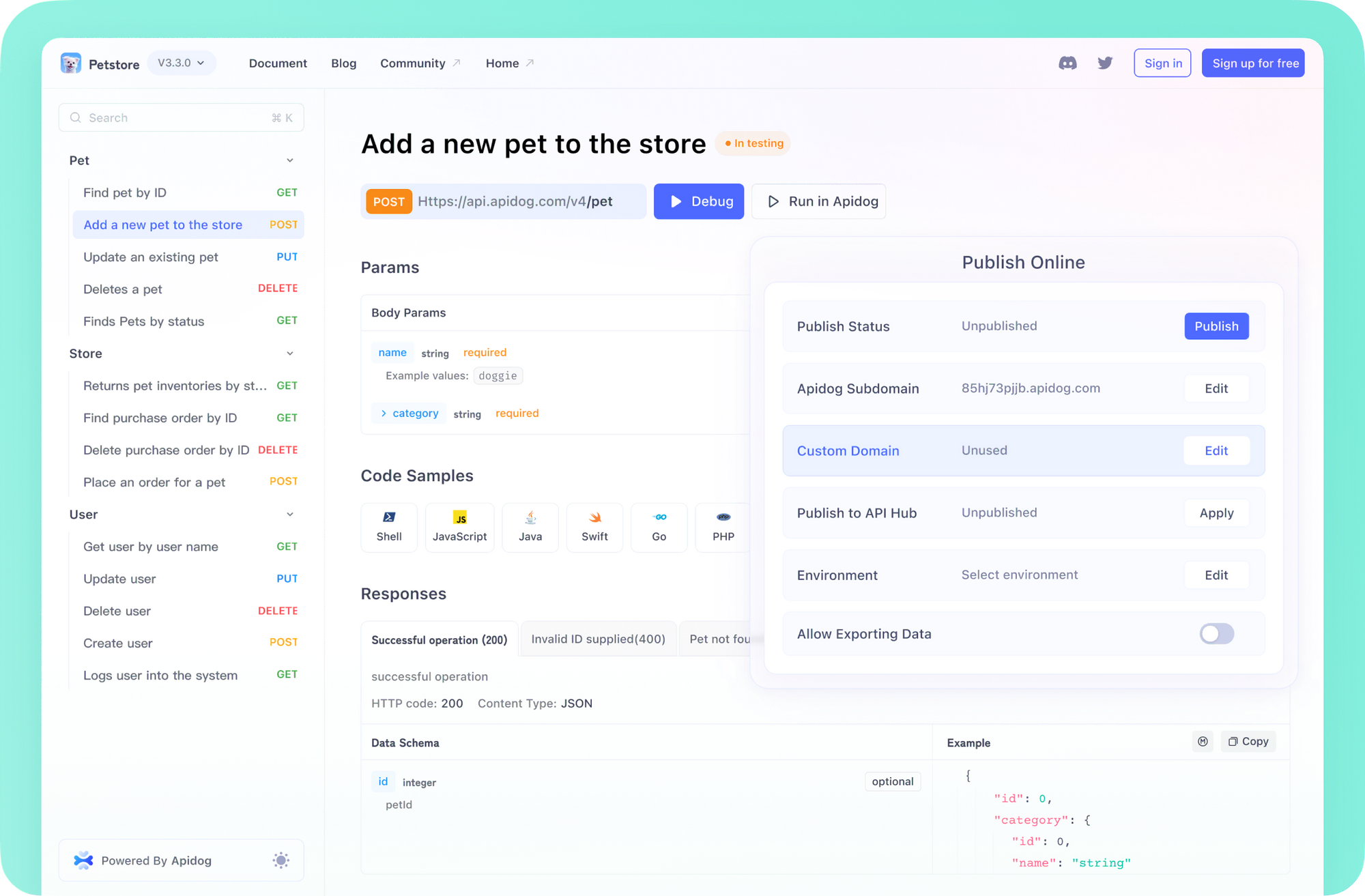