On April 13, 2025, SkyworkAI released the Skywork-OR1 (Open Reasoner 1) series, comprising three models: Skywork-OR1-Math-7B, Skywork-OR1-7B-Preview, and Skywork-OR1-32B-Preview.
- These models are trained using large-scale rule-based reinforcement learning specifically targeting mathematical and code reasoning capabilities.
- The models are built upon DeepSeek's distilled architectures: the 7B variants use DeepSeek-R1-Distill-Qwen-7B as their base, while the 32B model builds upon DeepSeek-R1-Distill-Qwen-32B.
Want an integrated, All-in-One platform for your Developer Team to work together with maximum productivity?
Apidog delivers all your demans, and replaces Postman at a much more affordable price!
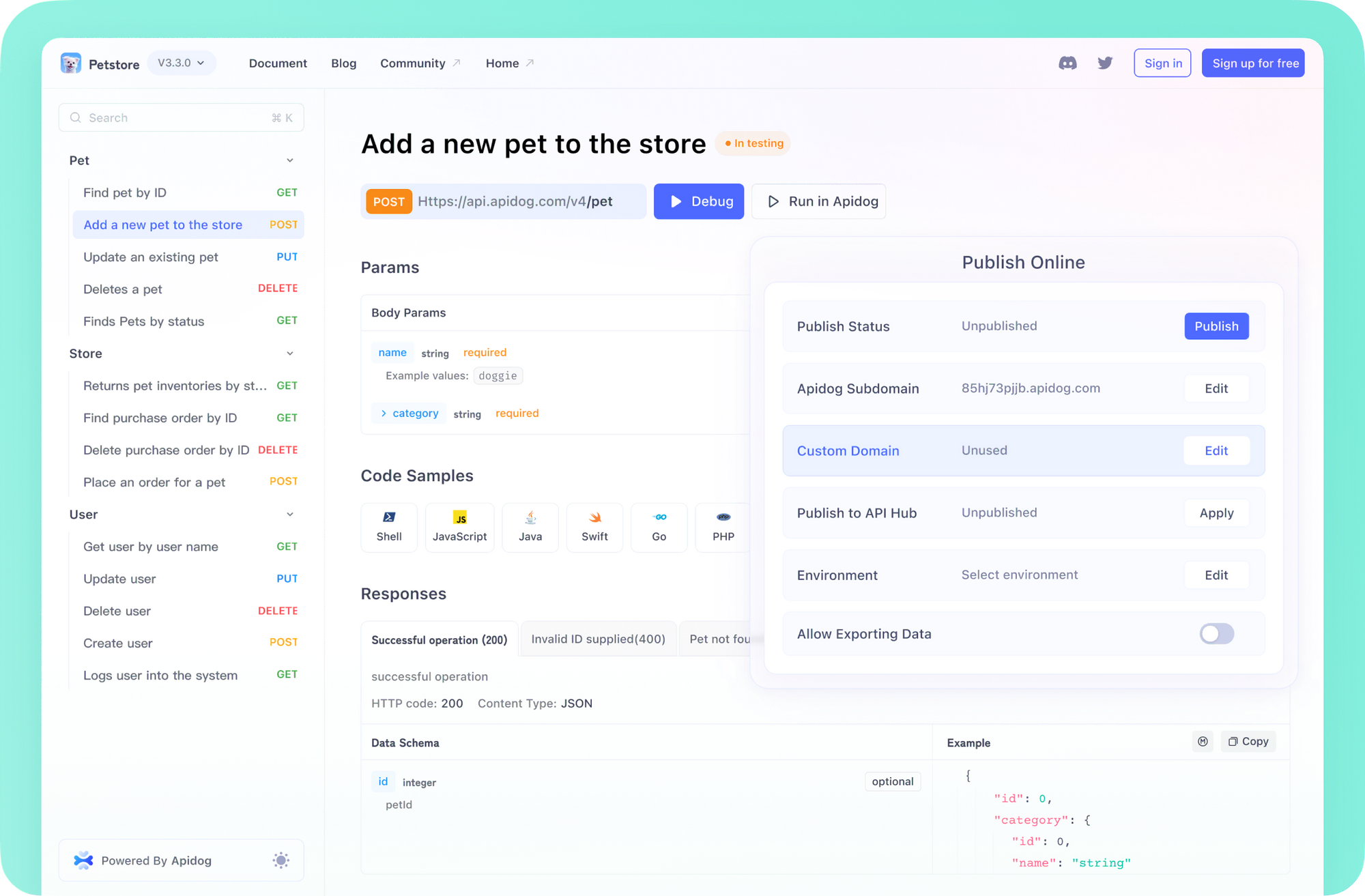
Skywork-OR1-32B: Not Just Another Open Source Reasoning Model
The Skywork-OR1-32B-Preview model contains 32.8 billion parameters and utilizes the BF16 tensor type for numerical precision. The model is distributed in the safetensors format and is based on the Qwen2 architecture. According to the model repository, it maintains the same architecture as the DeepSeek-R1-Distill-Qwen-32B base model, but with specialized training for mathematical and coding reasoning tasks.
Let's take a look at some of the Skywork model families' basic technical info:
Skywork-OR1-32B-Preview
- Parameter count: 32.8 billion
- Base model: DeepSeek-R1-Distill-Qwen-32B
- Tensor type: BF16
- Specialization: General-purpose reasoning
- Key performance:
- AIME24: 79.7 (Avg@32)
- AIME25: 69.0 (Avg@32)
- LiveCodeBench: 63.9 (Avg@4)
The 32B model demonstrates a 6.8-point improvement on AIME24 and a 10.0-point improvement on AIME25 over its base model. It achieves parameter efficiency by delivering performance comparable to the 671B parameter DeepSeek-R1 with only 4.9% of the parameters.
Skywork-OR1-Math-7B
- Parameter count: 7.62 billion
- Base model: DeepSeek-R1-Distill-Qwen-7B
- Tensor type: BF16
- Specialization: Mathematical reasoning
- Key performance:
- AIME24: 69.8 (Avg@32)
- AIME25: 52.3 (Avg@32)
- LiveCodeBench: 43.6 (Avg@4)
The model outperforms the base DeepSeek-R1-Distill-Qwen-7B significantly on mathematical tasks (69.8 vs. 55.5 on AIME24, 52.3 vs. 39.2 on AIME25), demonstrating the effectiveness of the specialized training approach.
Skywork-OR1-7B-Preview
- Parameter count: 7.62 billion
- Base model: DeepSeek-R1-Distill-Qwen-7B
- Tensor type: BF16
- Specialization: General-purpose reasoning
- Key performance:
- AIME24: 63.6 (Avg@32)
- AIME25: 45.8 (Avg@32)
- LiveCodeBench: 43.9 (Avg@4)
While showing less mathematical specialization than the Math-7B variant, this model offers better balanced performance between mathematical and coding tasks.
Traning Dataset of Skywork-OR1-32B
The Skywork-OR1 training dataset contains:
- 110,000 verifiable and diverse mathematical problems
- 14,000 coding questions
- All sourced from open-source datasets
Data Processing Pipeline
- Model-aware Difficulty Estimation: Each problem undergoes difficulty scoring relative to the model's current capabilities, allowing for targeted training.
- Quality Assessment: Rigorous filtering is applied prior to training to ensure dataset quality.
- Offline and Online Filtering: A two-stage filtering process is implemented to:
- Remove suboptimal examples before training (offline)
- Dynamically adjust problem selection during training (online)
4. Rejection Sampling: This technique is employed to control the distribution of training examples, helping maintain an optimal learning curve.
Advanced Reinforcement Learning Training Pipeline
The models utilize a customized version of GRPO (Generative Reinforcement via Policy Optimization) with several technical enhancements:
- Multi-stage Training Pipeline: The training proceeds through distinct phases, each building on previously acquired capabilities. The GitHub repository includes a graph plotting AIME24 scores against training steps, demonstrating clear performance improvements at each stage.
- Adaptive Entropy Control: This technique dynamically adjusts the exploration-exploitation trade-off during training, encouraging broader exploration while maintaining convergence stability.
- Custom Fork of VERL Framework: The models are trained using a modified version of the VERL project, specifically adapted for reasoning tasks.
You can read the full paper here.
Skywork-OR1-32B Benchmarks
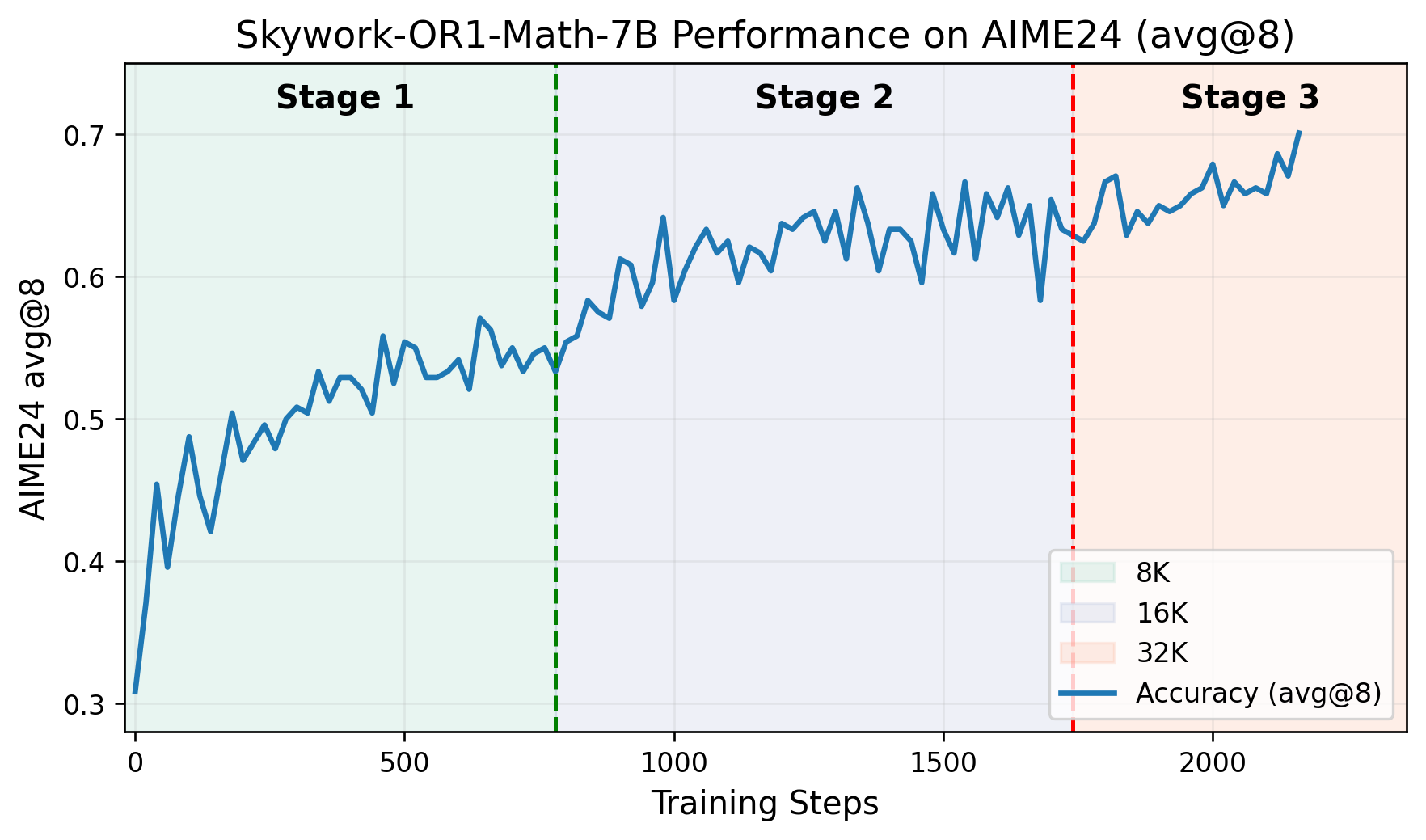
Technical specifications:
- Parameter count: 32.8 billion
- Tensor type: BF16
- Model format: Safetensors
- Architecture family: Qwen2
- Base model: DeepSeek-R1-Distill-Qwen-32B
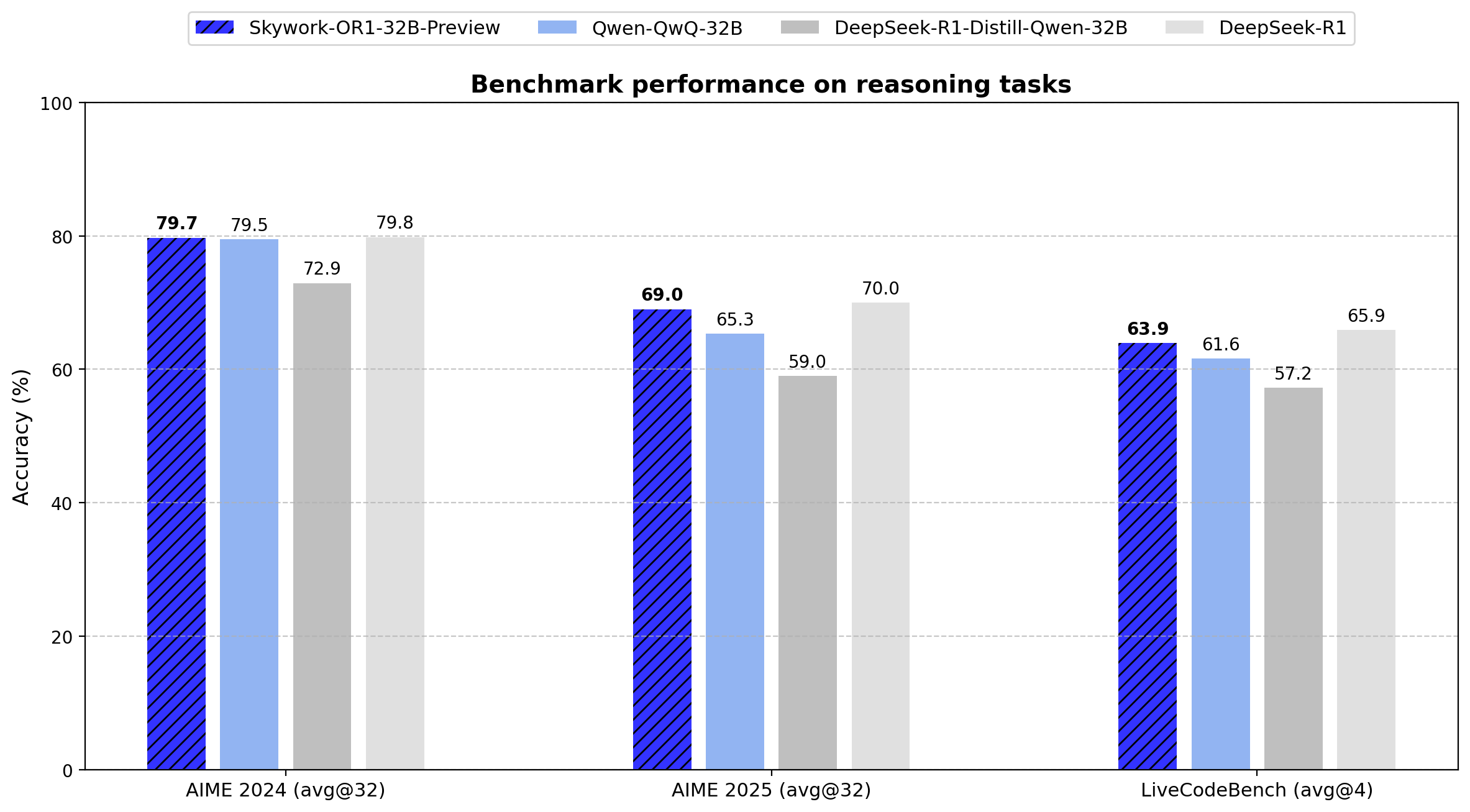
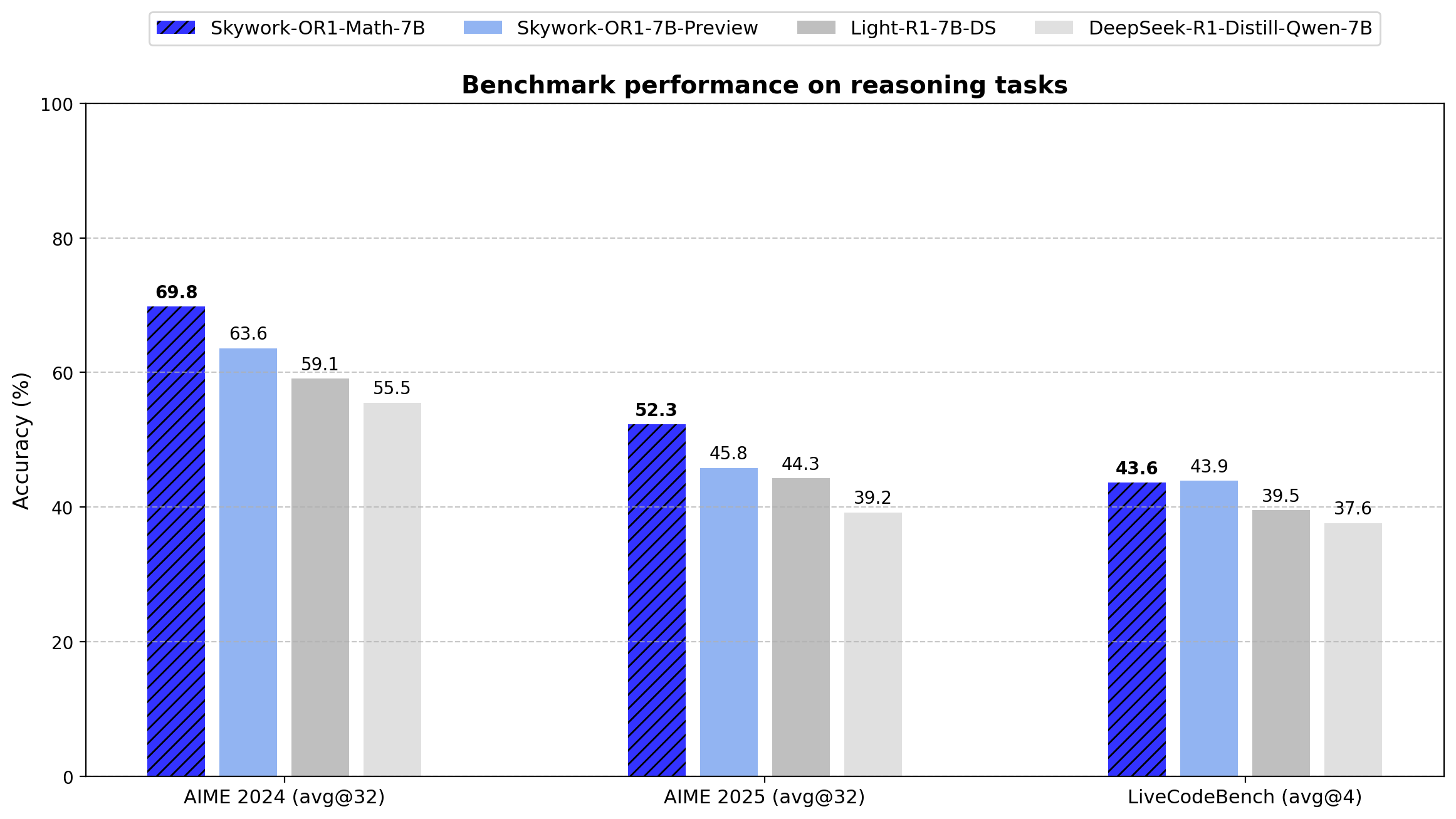
The Skywork-OR1 series introduces Avg@K as their primary evaluation metric instead of the conventional Pass@1. This metric calculates average performance across multiple independent attempts (32 for AIME tests, 4 for LiveCodeBench), reducing variance and providing a more reliable measure of reasoning consistency.
Below are the exact benchmark results for all models in the series:
Model | AIME24 (Avg@32) | AIME25 (Avg@32) | LiveCodeBench (8/1/24-2/1/25) (Avg@4) |
---|---|---|---|
DeepSeek-R1-Distill-Qwen-7B | 55.5 | 39.2 | 37.6 |
Light-R1-7B-DS | 59.1 | 44.3 | 39.5 |
DeepSeek-R1-Distill-Qwen-32B | 72.9 | 59.0 | 57.2 |
TinyR1-32B-Preview | 78.1 | 65.3 | 61.6 |
QwQ-32B | 79.5 | 65.3 | 61.6 |
DeepSeek-R1 | 79.8 | 70.0 | 65.9 |
Skywork-OR1-Math-7B | 69.8 | 52.3 | 43.6 |
Skywork-OR1-7B-Preview | 63.6 | 45.8 | 43.9 |
Skywork-OR1-32B-Preview | 79.7 | 69.0 | 63.9 |
The data shows that Skywork-OR1-32B-Preview performs at near-parity with DeepSeek-R1 (79.7 vs. 79.8 on AIME24, 69.0 vs. 70.0 on AIME25, and 63.9 vs. 65.9 on LiveCodeBench), despite the latter having 20 times more parameters (671B vs. 32.8B).
The Skywork-OR1 models can be implemented using the following technical specifications:
How to Test the Skywork-OR1 Models
Here are the Skywork-OR1-32B, Skywork-OR1-7B, and Skywork-OR1-Math-7B Hugging Face model cards:
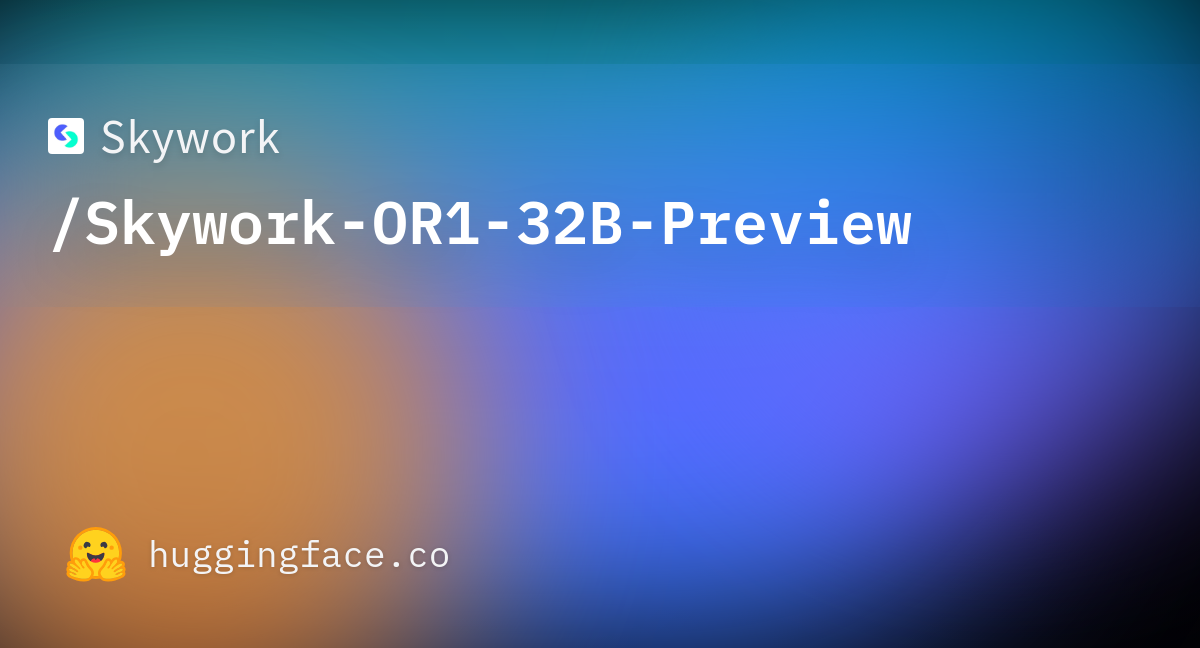
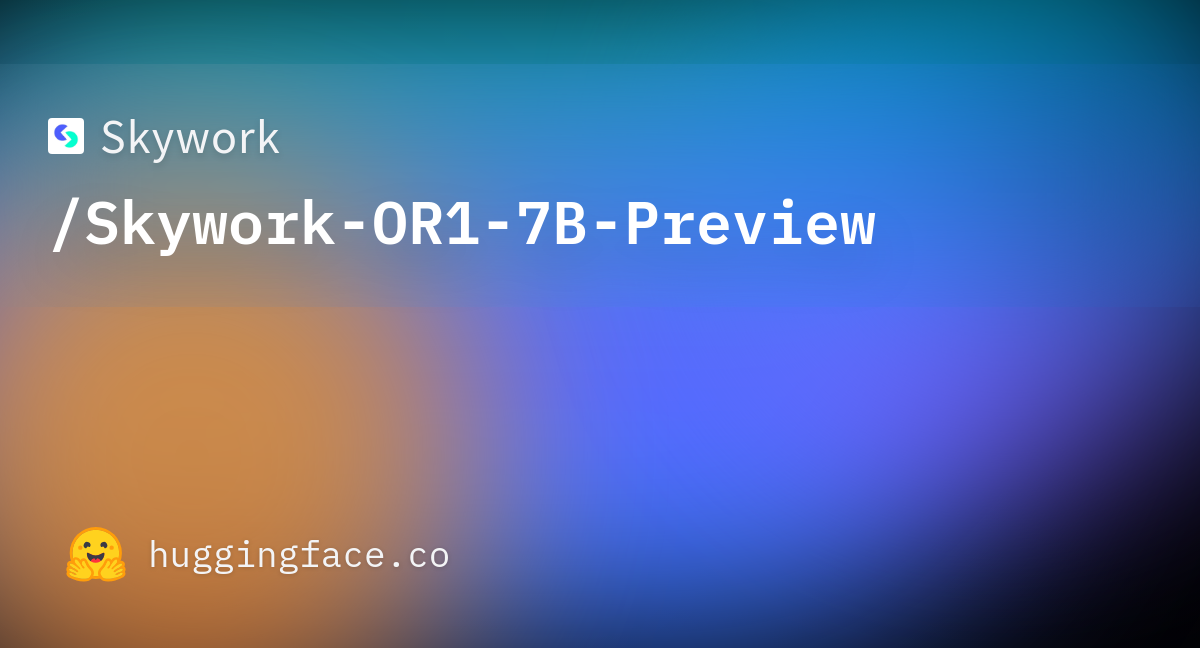
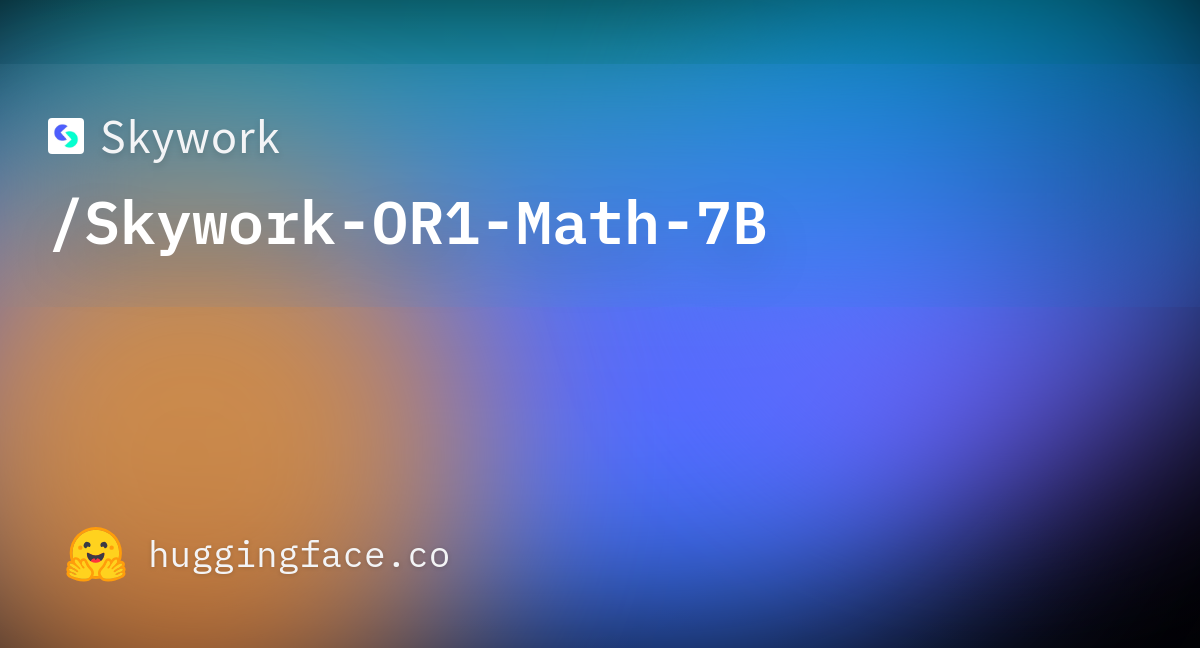
To run the Evaluation Scripts, take the following steps. First:
Docker Environment:
docker pull whatcanyousee/verl:vemlp-th2.4.0-cu124-vllm0.6.3-ray2.10-te2.0-megatron0.11.0-v0.0.6
docker run --runtime=nvidia -it --rm --shm-size=10g --cap-add=SYS_ADMIN -v <path>:<path> image:tag
Conda Environment Setup:
conda create -n verl python==3.10
conda activate verl
pip3 install torch==2.4.0 --index-url <https://download.pytorch.org/whl/cu124>
pip3 install flash-attn --no-build-isolation
git clone <https://github.com/SkyworkAI/Skywork-OR1.git>
cd Skywork-OR1
pip3 install -e .
For reproducing AIME24 evaluation:
MODEL_PATH=Skywork/Skywork-OR1-32B-Preview \\\\
DATA_PATH=or1_data/eval/aime24.parquet \\\\
SAMPLES=32 \\\\
TASK_NAME=Aime24_Avg-Skywork_OR1_Math_7B \\\\
bash ./or1_script/eval/eval_32b.sh
For AIME25 evaluation:
MODEL_PATH=Skywork/Skywork-OR1-Math-7B \\\\
DATA_PATH=or1_data/eval/aime25.parquet \\\\
SAMPLES=32 \\\\
TASK_NAME=Aime25_Avg-Skywork_OR1_Math_7B \\\\
bash ./or1_script/eval/eval_7b.sh
For LiveCodeBench evaluation:
MODEL_PATH=Skywork/Skywork-OR1-Math-7B \\\\
DATA_PATH=or1_data/eval/livecodebench/livecodebench_2408_2502.parquet \\\\
SAMPLES=4 \\\\
TASK_NAME=LiveCodeBench_Avg-Skywork_OR1_Math_7B \\\\
bash ./or1_script/eval/eval_7b.sh
The current Skywork-OR1 models are labeled as "Preview" versions, with final releases scheduled to be available within two weeks of the initial announcement. The developers have indicated that additional technical documentation will be released, including:
- A comprehensive technical report detailing the training methodology
- The Skywork-OR1-RL-Data dataset
- Additional training scripts
The GitHub repository notes that the training scripts are "currently being organized and will be available in 1-2 days."
Conclusion: Technical Assessment of Skywork-OR1-32B
The Skywork-OR1-32B-Preview model represents a significant advancement in parameter-efficient reasoning models. With 32.8 billion parameters, it achieves performance metrics nearly identical to the 671 billion parameter DeepSeek-R1 model across multiple benchmarks.
Though not verified yet, these results indicate that for practical applications requiring advanced reasoning capabilities, the Skywork-OR1-32B-Preview offers a viable alternative to significantly larger models, with substantially reduced computational requirements.
Additionally, the open-source nature of these models, along with their evaluation scripts and forthcoming training data, provides valuable technical resources for researchers and practitioners working on reasoning capabilities in language models.
Want an integrated, All-in-One platform for your Developer Team to work together with maximum productivity?
Apidog delivers all your demans, and replaces Postman at a much more affordable price!
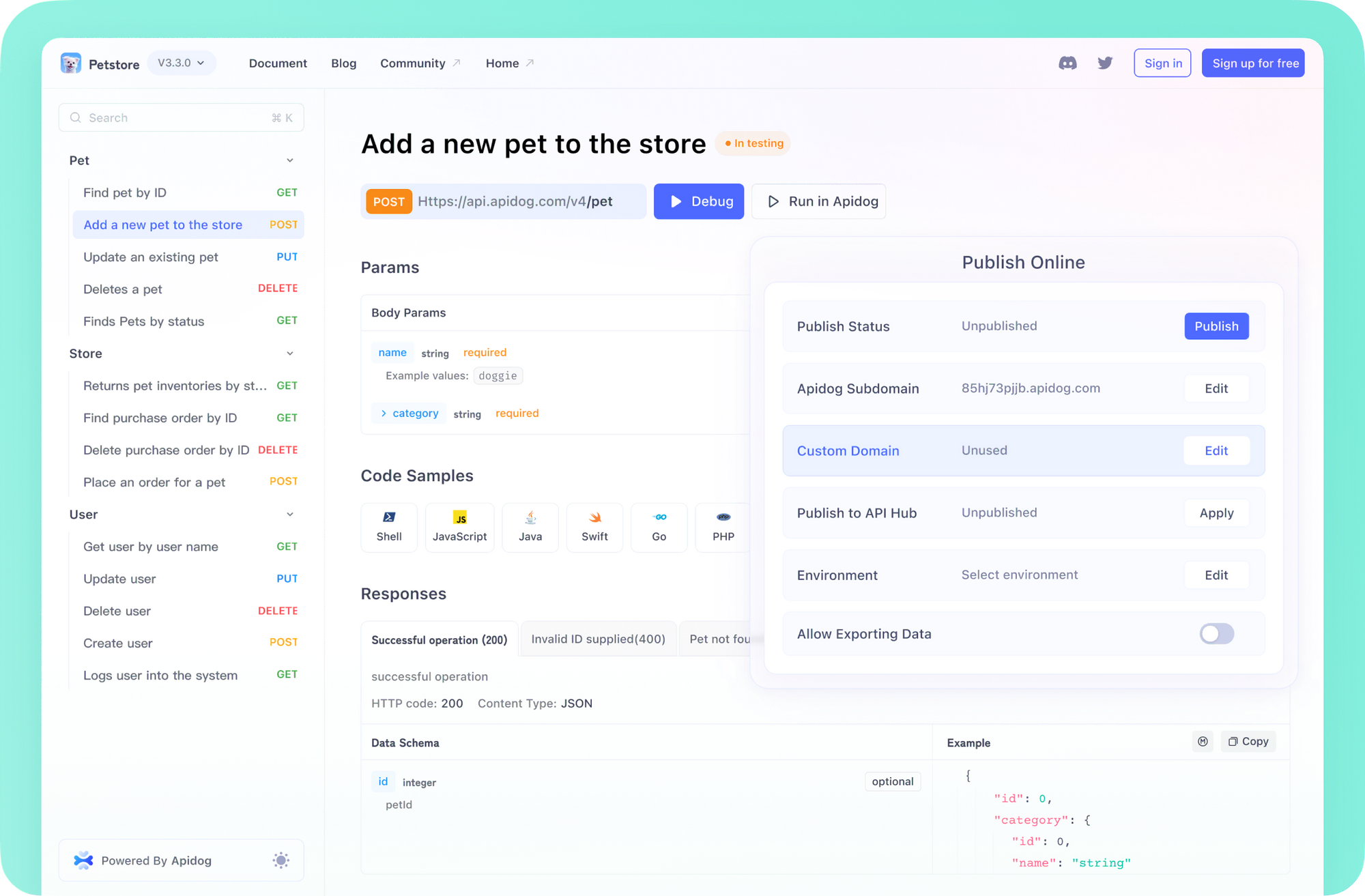