The landscape of large language models (LLMs) is evolving at breakneck speed. Models are becoming more powerful, capable, and, importantly, more accessible. The Qwen team recently unveiled Qwen3, their latest generation of LLMs, boasting impressive performance across various benchmarks, including coding, math, and general reasoning. With flagship models like the Mixture-of-Experts (MoE) Qwen3-235B-A22B rivaling established giants and even smaller dense models like Qwen3-4B competing with previous-generation 72B parameter models, Qwen3 represents a significant leap forward.
A key aspect of this release is the open-weighting of several models, including two MoE variants (Qwen3-235B-A22B and Qwen3-30B-A3B) and six dense models ranging from 0.6B to 32B parameters. This openness invites developers, researchers, and enthusiasts to explore, utilize, and build upon these powerful tools. While cloud-based APIs offer convenience, the desire to run these sophisticated models locally is growing, driven by needs for privacy, cost control, customization, and offline accessibility.
Fortunately, the tooling ecosystem for local LLM execution has matured significantly. Two standout platforms simplifying this process are Ollama and vLLM. Ollama provides an incredibly user-friendly way to get started with various models, while vLLM offers a high-performance serving solution optimized for throughput and efficiency, especially for larger models. This article will guide you through understanding Qwen3 and setting up these powerful models on your local machine using both Ollama and vLLM.
Want an integrated, All-in-One platform for your Developer Team to work together with maximum productivity?
Apidog delivers all your demans, and replaces Postman at a much more affordable price!
What is Qwen 3 and Benchmarks
Qwen3 represents the third generation of large language models (LLMs) developed by the Qwen team, released in April 2025. This iteration signifies a substantial advancement over previous versions, focusing on enhanced reasoning capabilities, efficiency through architectural innovations like Mixture-of-Experts (MoE), broader multilingual support, and improved performance across a wide range of benchmarks. The release included the open-weighting of several models under the Apache 2.0 license, promoting accessibility for research and development.
Qwen 3 Model Architecture and Variants, Explained
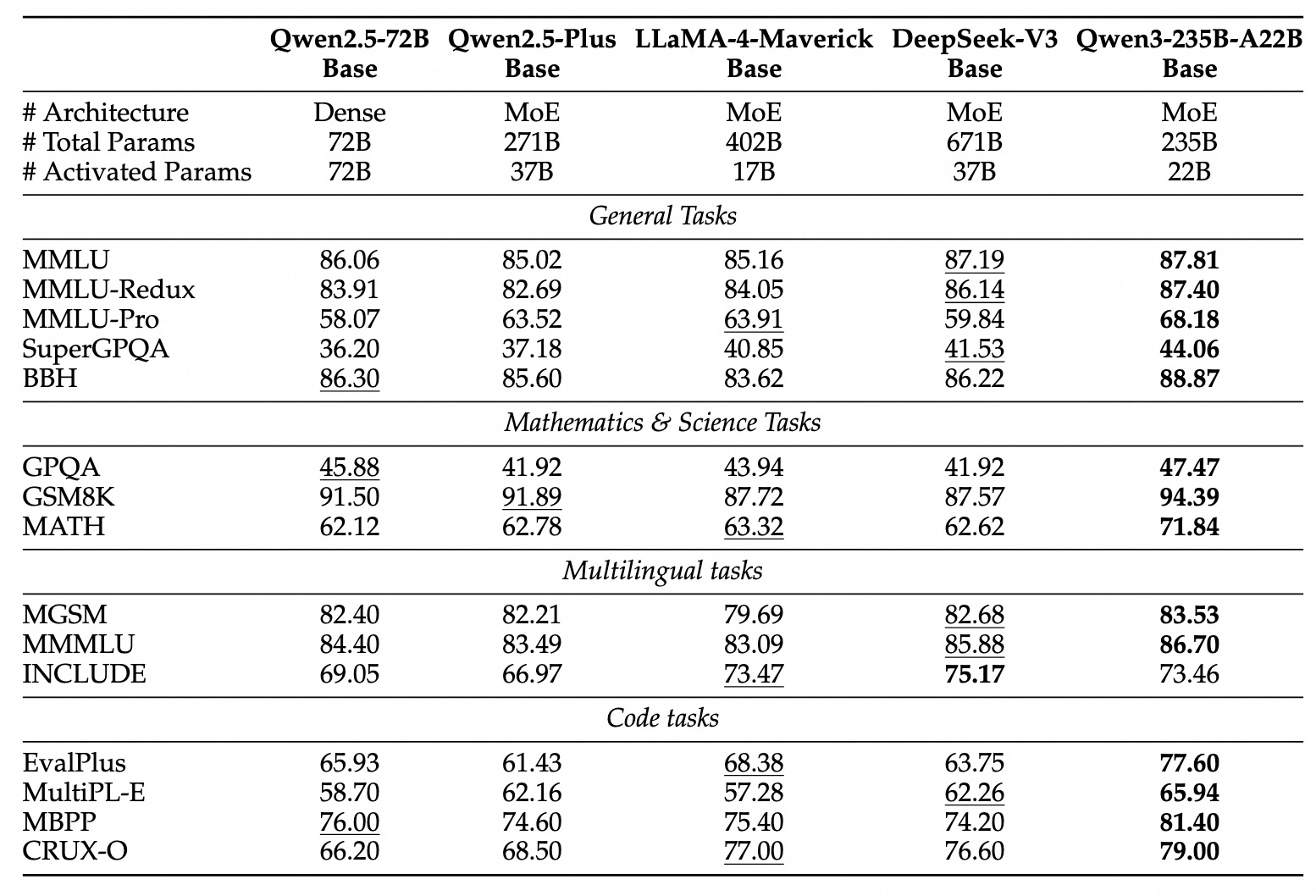
The Qwen3 family encompasses both traditional dense models and sparse MoE architectures, catering to diverse computational budgets and performance requirements.
Dense Models: These models utilize all their parameters during inference. Key architectural details include:
Model | Layers | Attention Heads (Query / Key-Value) | Tie Word Embeddings | Max Context Length |
---|---|---|---|---|
Qwen3-0.6B | 28 | 16 / 8 | Yes | 32,768 tokens (32K) |
Qwen3-1.7B | 28 | 16 / 8 | Yes | 32,768 tokens (32K) |
Qwen3-4B | 36 | 32 / 8 | Yes | 32,768 tokens (32K) |
Qwen3-8B | 36 | 32 / 8 | No | 131,072 tokens (128K) |
Qwen3-14B | 40 | 40 / 8 | No | 131,072 tokens (128K) |
Qwen3-32B | 64 | 64 / 8 | No | 131,072 tokens (128K) |
Note: Grouped-Query Attention (GQA) is employed in all models, indicated by the differing number of Query and Key-Value heads.
Mixture-of-Experts (MoE) Models: These models leverage sparsity by activating only a subset of "expert" Feed-Forward Networks (FFNs) for each token during inference. This allows for a large total parameter count while maintaining computational costs closer to smaller dense models.
Model | Layers | Attention Heads (Query / Key-Value) | # Experts (Total / Activated) | Max Context Length |
---|---|---|---|---|
Qwen3-30B-A3B | 48 | 32 / 4 | 128 / 8 | 131,072 tokens (128K) |
Qwen3-235B-A22B | 94 | 64 / 4 | 128 / 8 | 131,072 tokens (128K) |
Note: Both MoE models utilize 128 total experts but activate only 8 per token, significantly reducing the computational load compared to a dense model of equivalent size.
Qwen 3 Key Technical Features
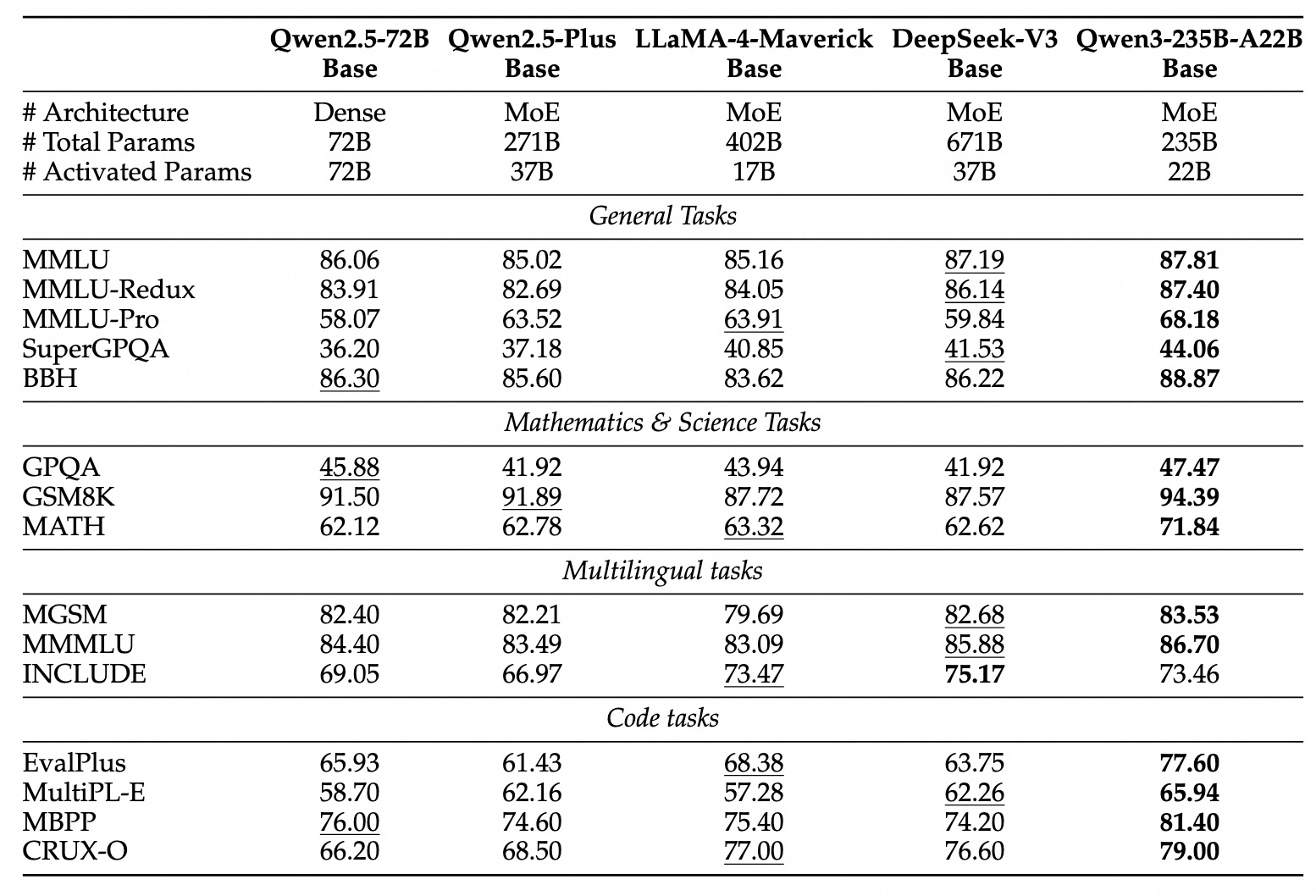
Hybrid Thinking Modes: A distinctive feature of Qwen3 is its ability to operate in two distinct modes, controllable by the user:
- Thinking Mode (Default): The model performs internal, step-by-step reasoning (Chain-of-Thought style) before generating the final response. This latent thought process is encapsulated, often marked by special tokens (e.g., outputting
<think>...</think>
content before the final answer when using specific framework configurations). This mode enhances performance on complex tasks requiring logical deduction, mathematical reasoning, or planning. It allows for scalable performance improvements directly correlated with the allocated computational reasoning budget. - Non-Thinking Mode: The model generates a direct response without the explicit internal reasoning phase, optimizing for speed and reduced computational cost on simpler queries.
Users can dynamically switch between these modes, potentially on a turn-by-turn basis in multi-turn conversations using tags like/think
and/no_think
in their prompts (framework permitting), enabling fine-grained control over the trade-off between latency/cost and reasoning depth.
Extensive Multilingual Support: Qwen3 models are pre-trained on a diverse corpus enabling support for 119 languages and dialects across major language families (Indo-European, Sino-Tibetan, Afro-Asiatic, Austronesian, Dravidian, Turkic, etc.), making them suitable for a wide array of global applications.
Advanced Training Methodology:
- Pre-training: Models were pre-trained on a large-scale dataset comprising trillions of tokens. The final pre-training stage involved using high-quality long-context data to extend the effective context window up to 32K tokens initially, with further extensions to 128K for larger models.
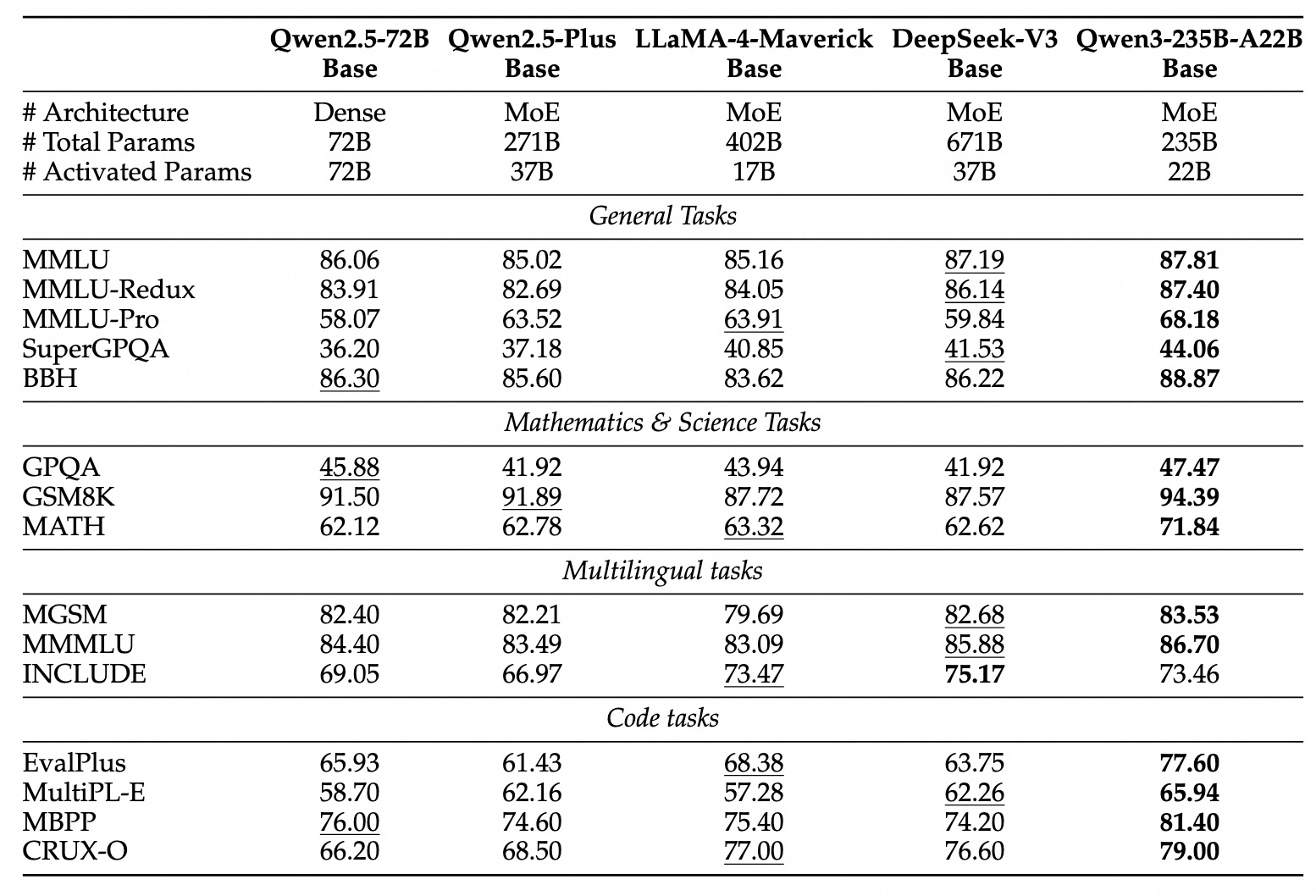
- Post-training: A sophisticated four-stage pipeline was employed to imbue the models with instruction-following capabilities, reasoning skills, and the hybrid thinking mechanism:
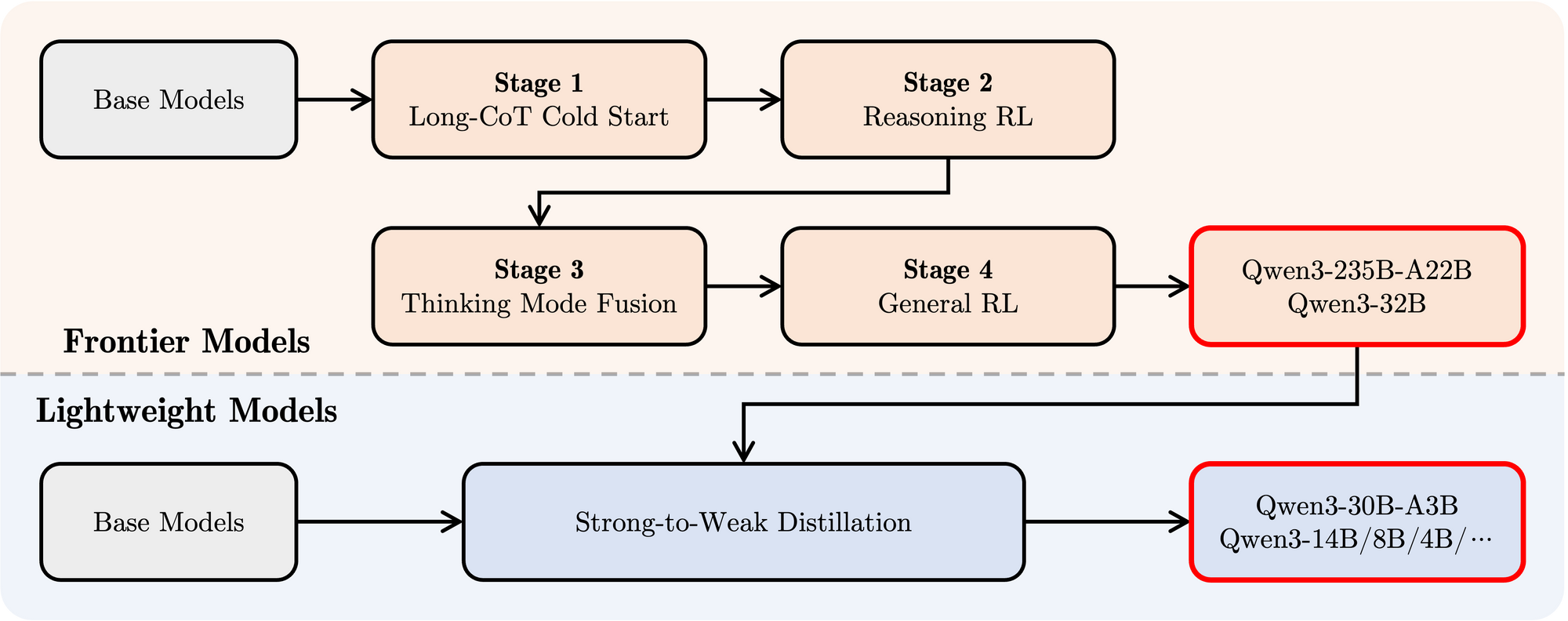
- Long CoT Cold Start: Supervised fine-tuning (SFT) on diverse long Chain-of-Thought (CoT) data spanning mathematics, coding, logical reasoning, and STEM to build foundational reasoning abilities.
- Reasoning-based Reinforcement Learning (RL): Scaling up computational resources for RL using rule-based rewards to enhance exploration and exploitation specifically for reasoning tasks.
- Thinking Mode Fusion: Integrating non-thinking capabilities by fine-tuning the reasoning-enhanced model on a mix of long CoT data and standard instruction-tuning data generated by the Stage 2 model. This blends deep reasoning with rapid response generation.
- General RL: Applying RL across numerous general-domain tasks (instruction following, format adherence, agent capabilities) to refine overall behavior and mitigate undesired outputs.
Qwen 3 Benchmark Performance
Qwen3 demonstrates highly competitive performance against other leading contemporary models:
Flagship MoE: The Qwen3-235B-A22B
model achieves results comparable to top-tier models such as DeepSeek-R1, Google's o1 and o3-mini, Grok-3, and Gemini-2.5-Pro across various benchmarks evaluating coding, math, and general capabilities.
Smaller MoE: The Qwen3-30B-A3B
model significantly outperforms models like QwQ-32B, despite activating only a fraction (3B vs 32B) of the parameters during inference, highlighting the efficiency of the MoE architecture.
Dense Models: Due to architectural and training advancements, Qwen3 dense models generally match or exceed the performance of larger Qwen2.5 dense models. For example:
Qwen3-1.7B
≈Qwen2.5-3B
Qwen3-4B
≈Qwen2.5-7B
(and rivalsQwen2.5-72B-Instruct
in some aspects)Qwen3-8B
≈Qwen2.5-14B
Qwen3-14B
≈Qwen2.5-32B
Qwen3-32B
≈Qwen2.5-72B
Notably, Qwen3 dense base models show particularly strong performance improvements over their predecessors in STEM, coding, and reasoning tasks.
MoE Efficiency: Qwen3 MoE base models achieve performance comparable to significantly larger Qwen2.5 dense models while activating only ~10% of the parameters, leading to substantial savings in both training and inference compute.
These benchmark results underscore Qwen3's position as a state-of-the-art model family offering both high performance and, particularly with MoE variants, improved computational efficiency. The models are available through standard platforms like Hugging Face, ModelScope, and Kaggle, and are supported by popular deployment frameworks such as Ollama, vLLM, SGLang, LMStudio, and llama.cpp, facilitating their integration into various workflows and applications, including local execution.
How to Run Qwen 3 Locally with Ollama
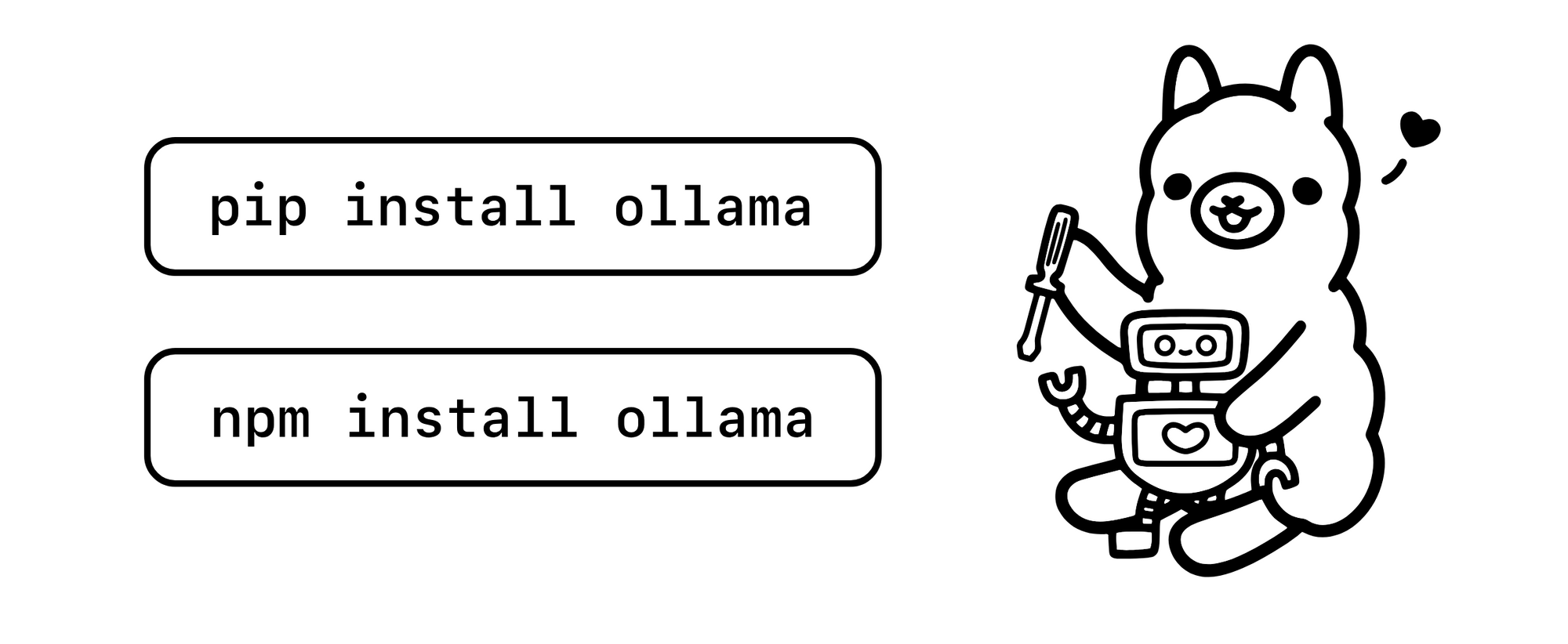
Ollama has gained immense popularity for its simplicity in downloading, managing, and running LLMs locally. It abstracts away much of the complexity, providing a command-line interface and an API server.
1. Installation:
Installing Ollama is typically straightforward. Visit the official Ollama website (ollama.com
) and follow the download instructions for your operating system (macOS, Linux, Windows).
2. Pulling Qwen3 Models:
Ollama maintains a library of readily available models. To run a specific Qwen3 model, you use the ollama run
command. If the model isn't present locally, Ollama automatically downloads it. The Qwen team has made several Qwen3 variants available directly on the Ollama library.
You can find available Qwen3 tags on the Ollama website's Qwen3 page (e.g., ollama.com/library/qwen3
). Common tags might include:
qwen3:0.6b
qwen3:1.7b
qwen3:4b
qwen3:8b
qwen3:14b
qwen3:32b
qwen3:30b-a3b
(The smaller MoE model)
To run the 4B parameter model, for instance, simply open your terminal and type:
ollama run qwen3:4b
This command will download the model (if needed) and start an interactive chat session.
3. Interacting with the Model:
Once the ollama run
command is active, you can type your prompts directly into the terminal. Ollama also starts a local server (typically at http://localhost:11434
) that exposes an API compatible with the OpenAI standard. You can interact with this programmatically using tools like curl
or various client libraries in Python, JavaScript, etc.
4. Hardware Considerations:
Running LLMs locally requires substantial resources.
- RAM: Even smaller models (0.6B, 1.7B) require several gigabytes of RAM. Larger models (8B, 14B, 32B, 30B-A3B) need significantly more, often 16GB, 32GB, or even 64GB+, depending on the quantization level used by Ollama.
- VRAM (GPU): For acceptable performance, a dedicated GPU with ample VRAM is highly recommended. Ollama automatically utilizes compatible GPUs (NVIDIA, Apple Silicon). The amount of VRAM dictates the largest model you can comfortably run entirely on the GPU, which significantly speeds up inference.
- CPU: While Ollama can run models on the CPU, performance will be considerably slower than on a GPU.
Ollama is excellent for getting started quickly, local development, experimentation, and single-user chat applications, especially on consumer-grade hardware (within limits).
How to Run Ollama Locally with vLLM
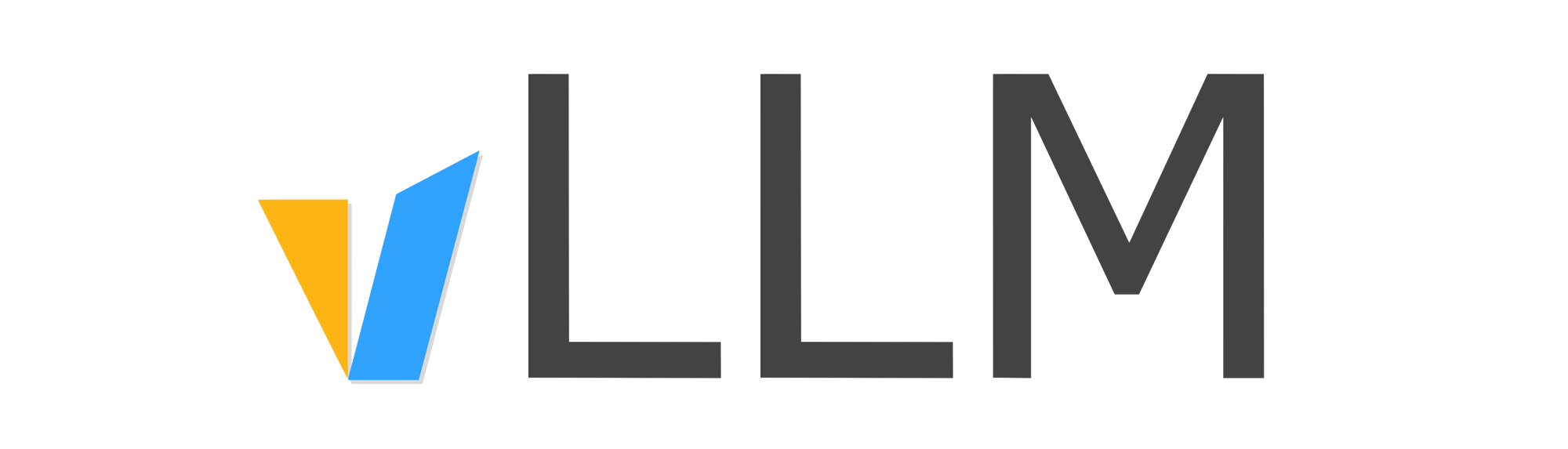
vLLM is a high-throughput LLM serving library that employs optimizations like PagedAttention to significantly improve inference speed and memory efficiency, making it ideal for demanding applications and serving larger models. The vLLM team provides excellent support for new architectures, including Day 0 support for Qwen3 upon its release.
1. Installation:
Install vLLM using pip. It's generally recommended to use a virtual environment:
pip install -U vllm
Ensure you have the necessary prerequisites, typically a compatible NVIDIA GPU with the appropriate CUDA toolkit installed. Refer to the vLLM documentation for specific requirements.
2. Serving Qwen3 Models:
vLLM uses the vllm serve
command to load a model and launch an OpenAI-compatible API server. The Qwen team and vLLM documentation provide guidance on running Qwen3.
Based on the information provided and common vLLM usage, here's how you might serve the large Qwen3-235B MoE model using FP8 quantization (for reduced memory usage) and tensor parallelism across 4 GPUs:
vllm serve Qwen/Qwen3-235B-A22B-FP8 \
--enable-reasoning \
--reasoning-parser deepseek_r1 \
--tensor-parallel-size 4
Let's break down this command:
Qwen/Qwen3-235B-A22B-FP8
: This is the model identifier, likely pointing to a Hugging Face repository location.FP8
indicates the use of 8-bit floating-point quantization, reducing the model's memory footprint compared to FP16 or BF16, which is crucial for such a large model.--enable-reasoning
: This flag is vital for activating Qwen3's hybrid thinking capabilities within vLLM.--reasoning-parser deepseek_r1
: Qwen3's thinking output has a specific format. vLLM requires a parser to handle this. The blog post indicates that for vLLM, thedeepseek_r1
parser should be used (while SGLang uses aqwen3
parser). This ensures vLLM can correctly interpret and potentially separate the thinking steps from the final response.--tensor-parallel-size 4
: This instructs vLLM to distribute the model's weights and computation across 4 GPUs. Tensor parallelism is essential for running models too large to fit on a single GPU. You would adjust this number based on your available GPUs.
You can adapt this command for other Qwen3 models (e.g., Qwen/Qwen3-30B-A3B
or Qwen/Qwen3-32B
) and adjust parameters like tensor-parallel-size
based on your hardware.
3. Interacting with the vLLM Server:
Once vllm serve
is running, it hosts an API server (defaulting to http://localhost:8000
) that mirrors the OpenAI API specification. You can interact with it using standard tools:
- curl:
curl http://localhost:8000/v1/completions \
-H "Content-Type: application/json" \
-d '{
"model": "Qwen/Qwen3-235B-A22B-FP8", # Use the model name you served
"prompt": "Explain the concept of Mixture-of-Experts in LLMs.",
"max_tokens": 150,
"temperature": 0.7
}'
- Python OpenAI Client:
from openai import OpenAI
# Point to the local vLLM server
client = OpenAI(base_url="http://localhost:8000/v1", api_key="EMPTY")
completion = client.completions.create(
model="Qwen/Qwen3-235B-A22B-FP8", # Use the model name you served
prompt="Write a short story about a robot discovering music.",
max_tokens=200
)
print(completion.choices[0].text)
4. Performance and Use Cases:
vLLM shines in scenarios requiring high throughput (many requests per second) and low latency. Its optimizations make it suitable for:
- Building applications powered by local LLMs.
- Serving models to multiple users concurrently.
- Deploying large models that necessitate multi-GPU setups.
- Production environments where performance is critical.
Testing Ollama Local API with Apidog
Apidog is an API testing tool that pairs well with Ollama’s API mode. It lets you send requests, view responses, and debug your Qwen 3 setup efficiently.
Here’s how to use Apidog with Ollama:
- Create a new API request:
- Endpoint:
http://localhost:11434/api/generate
- Send the request and monitor the response in Apidog’s real-time timeline.
- Use Apidog’s JSONPath extraction to parse responses automatically, a feature that outshines tools like Postman.
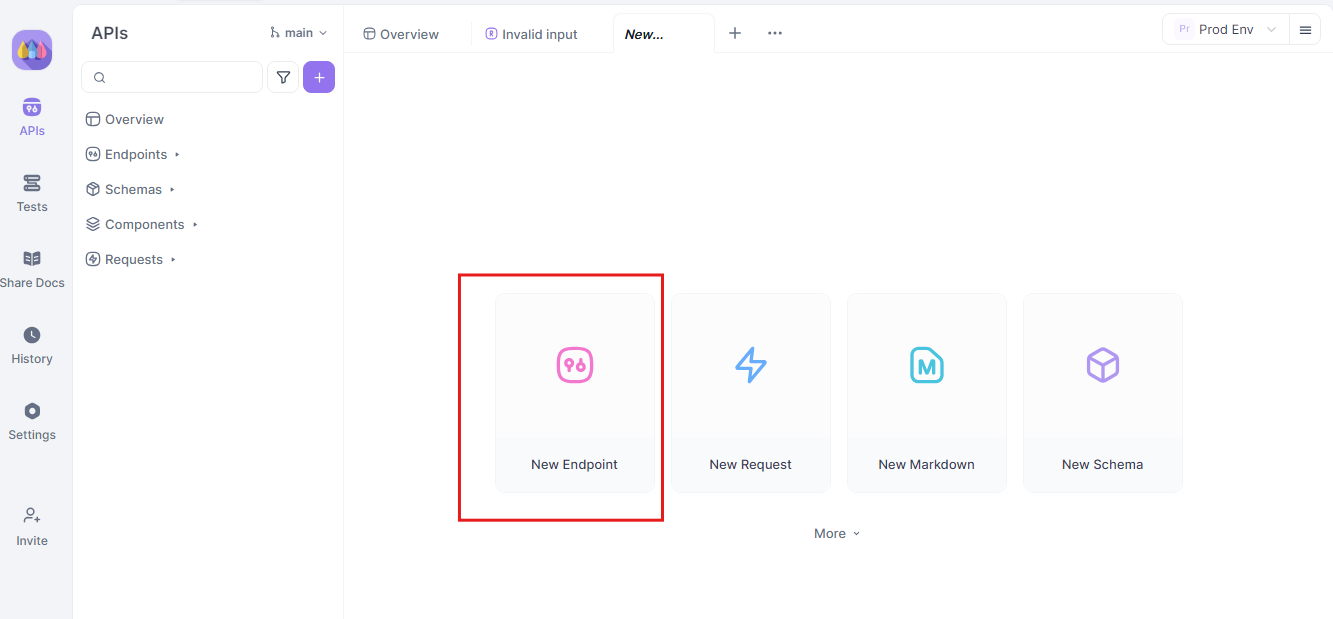


Streaming Responses:
- For real-time applications, enable streaming:
- Apidog’s Auto-Merge feature consolidates streamed messages, simplifying debugging.
curl http://localhost:11434/api/generate -d '{"model": "gemma3:4b-it-qat", "prompt": "Write a poem about AI.", "stream": true}'
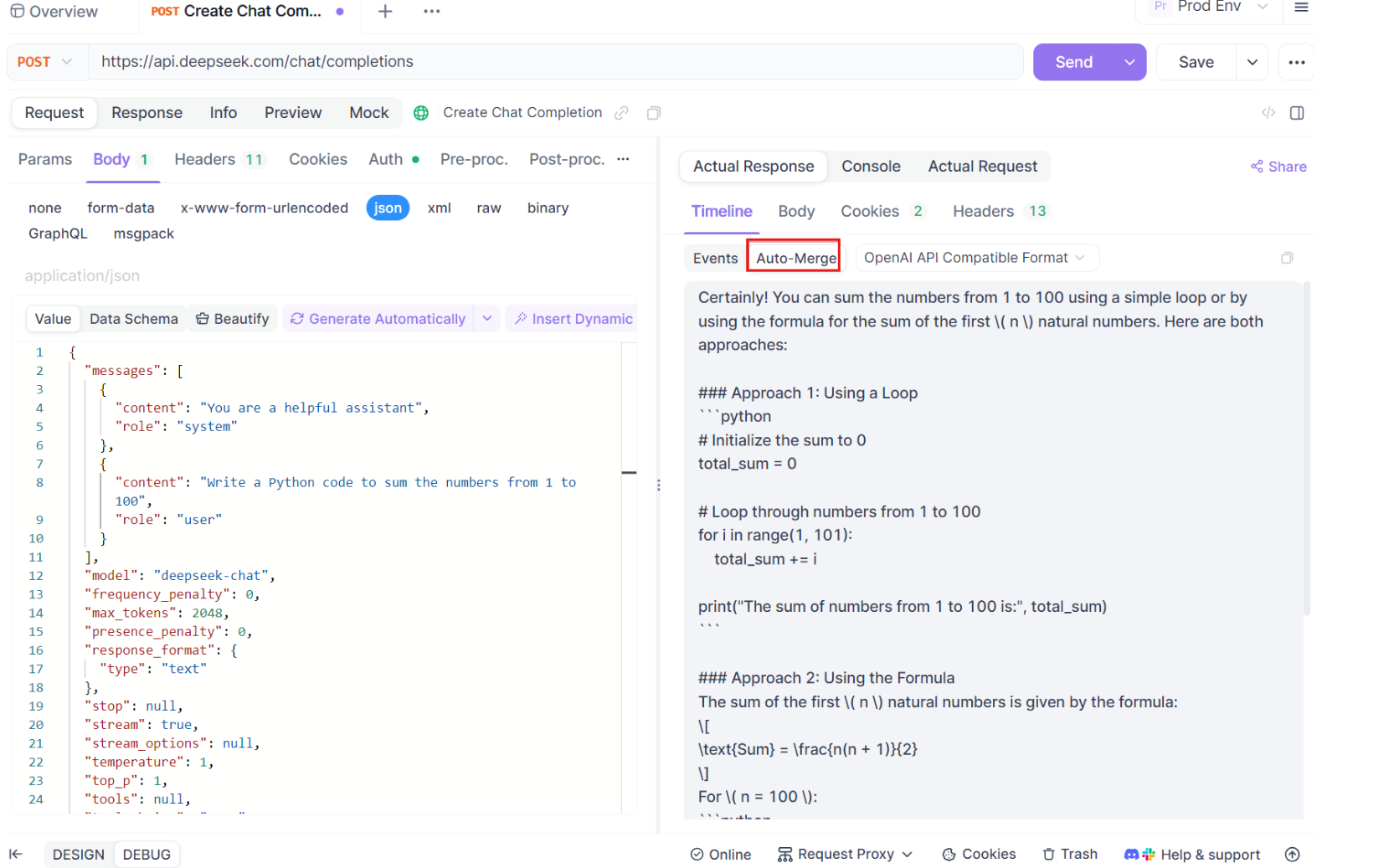
This process ensures your model works as expected, making Apidog a valuable addition.
Conclusion
The release of the powerful and diverse Qwen3 model family, combined with mature local execution tools like Ollama and vLLM, marks an exciting time for AI practitioners. Whether you prioritize the plug-and-play simplicity of Ollama for personal use and experimentation or the high-performance serving capabilities of vLLM for building robust applications, running state-of-the-art LLMs locally is more feasible than ever.
By bringing models like Qwen3-30B-A3B or even the larger dense variants onto your own hardware, you gain unprecedented control, privacy, and cost-effectiveness. You can leverage their advanced features, like hybrid thinking and extensive multilingual support, for innovative projects. As the hardware and software ecosystems continue to improve, the power of large language models will become increasingly democratized, moving from distant cloud servers right onto our local machines. Experiment with Qwen3 using Ollama and vLLM to experience the forefront of this local AI revolution.
Want an integrated, All-in-One platform for your Developer Team to work together with maximum productivity?
Apidog delivers all your demans, and replaces Postman at a much more affordable price!