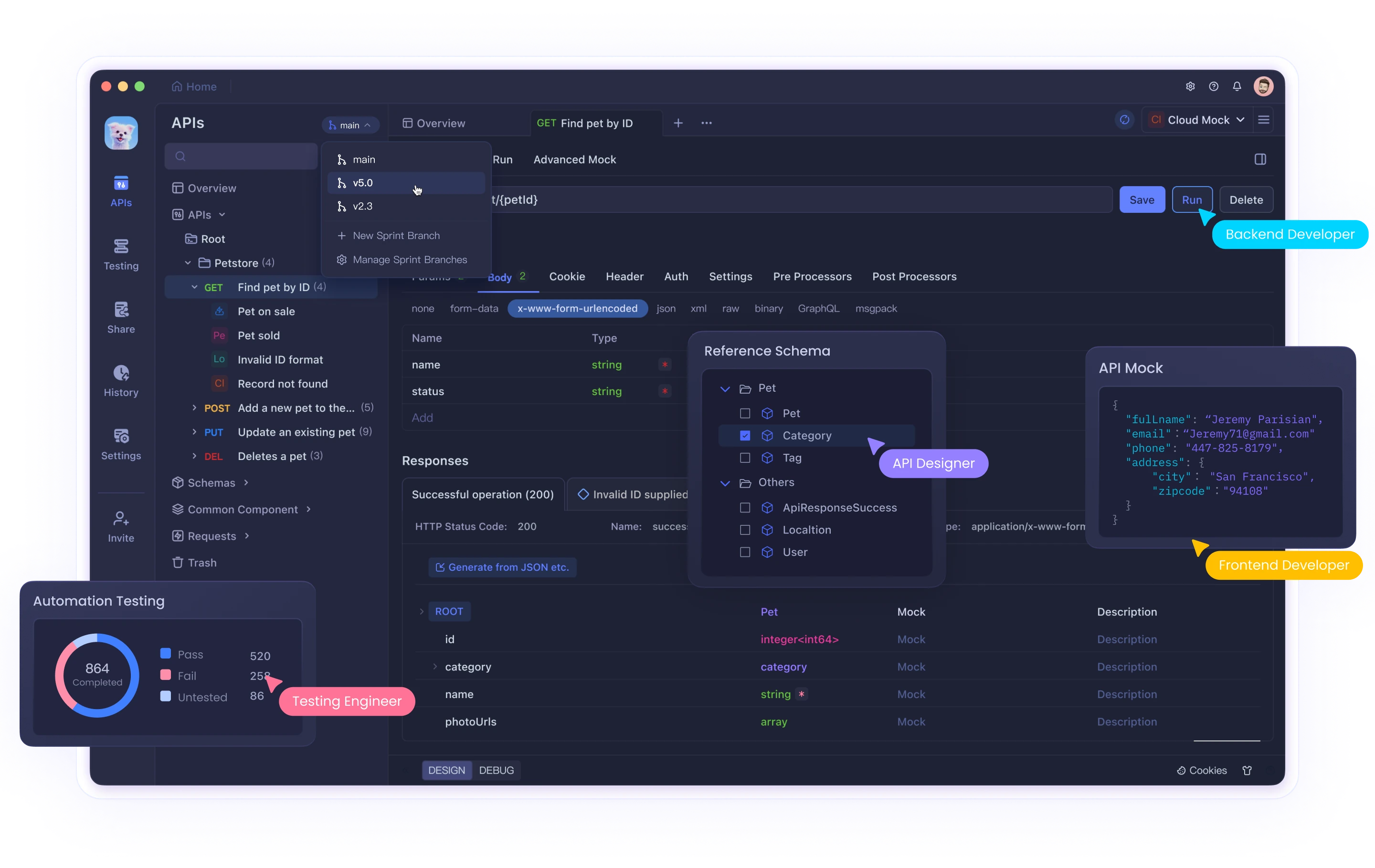
In the rapidly evolving world of artificial intelligence, a new milestone has been reached with the release of Qwen 2.5 Omni 7B. This revolutionary model from Alibaba Cloud represents a significant leap forward in multimodal AI, combining the ability to process and understand multiple forms of input while generating both text and speech outputs. Let's dive into what makes this model truly special and how it's reshaping our understanding of AI capabilities.
The True Meaning of "Omni" within Qwen 2.5 Omni 7B
The term "Omni" in Qwen 2.5 Omni 7B isn't just clever branding—it's a fundamental description of the model's capabilities. Unlike many multimodal models that excel in one or two data types, Qwen 2.5 Omni 7B is designed from the ground up to perceive and understand:
- Text (written language)
- Images (visual information)
- Audio (sounds and spoken language)
- Video (moving visual content with temporal dimension)
Voice Chat + Video Chat! Just in Qwen Chat (https://t.co/FmQ0B9tiE7)! You can now chat with Qwen just like making a phone call or making a video call! Check the demo in https://t.co/42iDe4j1Hs
— Qwen (@Alibaba_Qwen) March 26, 2025
What's more, we opensource the model behind all this, Qwen2.5-Omni-7B, under the… pic.twitter.com/LHQOQrl9Ha
What's even more impressive is that this model doesn't just take in these varied inputs—it can respond with both text and natural speech outputs in a streaming fashion. This "any-to-any" capability represents a significant advancement toward more natural and human-like AI interactions.
The Innovative Architecture of Qwen 2.5 Omni 7B: Explained
Thinker-Talker: A New Paradigm
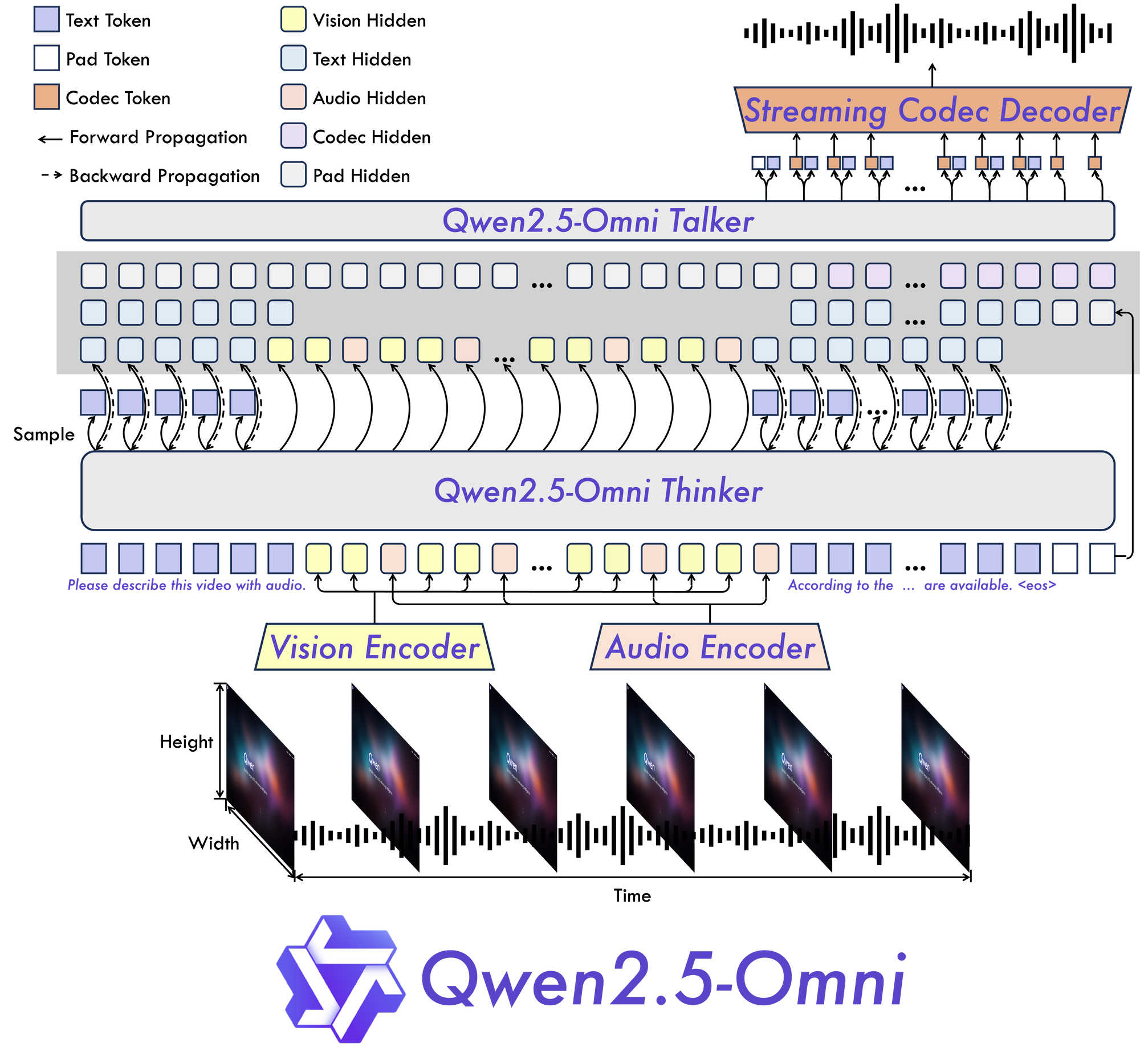
At the heart of Qwen 2.5 Omni 7B lies its foundational "Thinker-Talker" architecture. This novel design creates a model specifically built to be end-to-end multimodal, allowing for seamless processing across different types of information.
As the name suggests, this architecture separates the cognitive processing of information (thinking) from the generation of outputs (talking). This separation enables the model to effectively manage the inherent complexities of multimodal data and produce appropriate responses in multiple formats.
TMRoPE: Solving the Temporal Alignment Challenge
One of the most significant innovations in Qwen 2.5 Omni 7B is its Time-aligned Multimodal RoPE (TMRoPE) mechanism. This breakthrough tackles one of the most challenging aspects of multimodal AI: synchronizing temporal data from different sources.
When processing video and audio simultaneously, the model needs to understand how visual events align with corresponding sounds or speech. For example, matching a person's lip movements with their spoken words requires precise temporal alignment. TMRoPE provides the sophisticated framework for achieving this synchronization, enabling the model to build a coherent understanding of multimodal inputs that unfold over time.
Designed for Real-Time Interaction
Qwen 2.5 Omni 7B was built with real-time applications in mind. The architecture supports low-latency streaming, allowing for chunked input processing and immediate output generation. This makes it ideal for applications requiring responsive interactions, such as voice assistants, live video analysis, or real-time translation services.
Qwen 2.5 Omni 7B Performance: Benchmarks Speaks for Itself
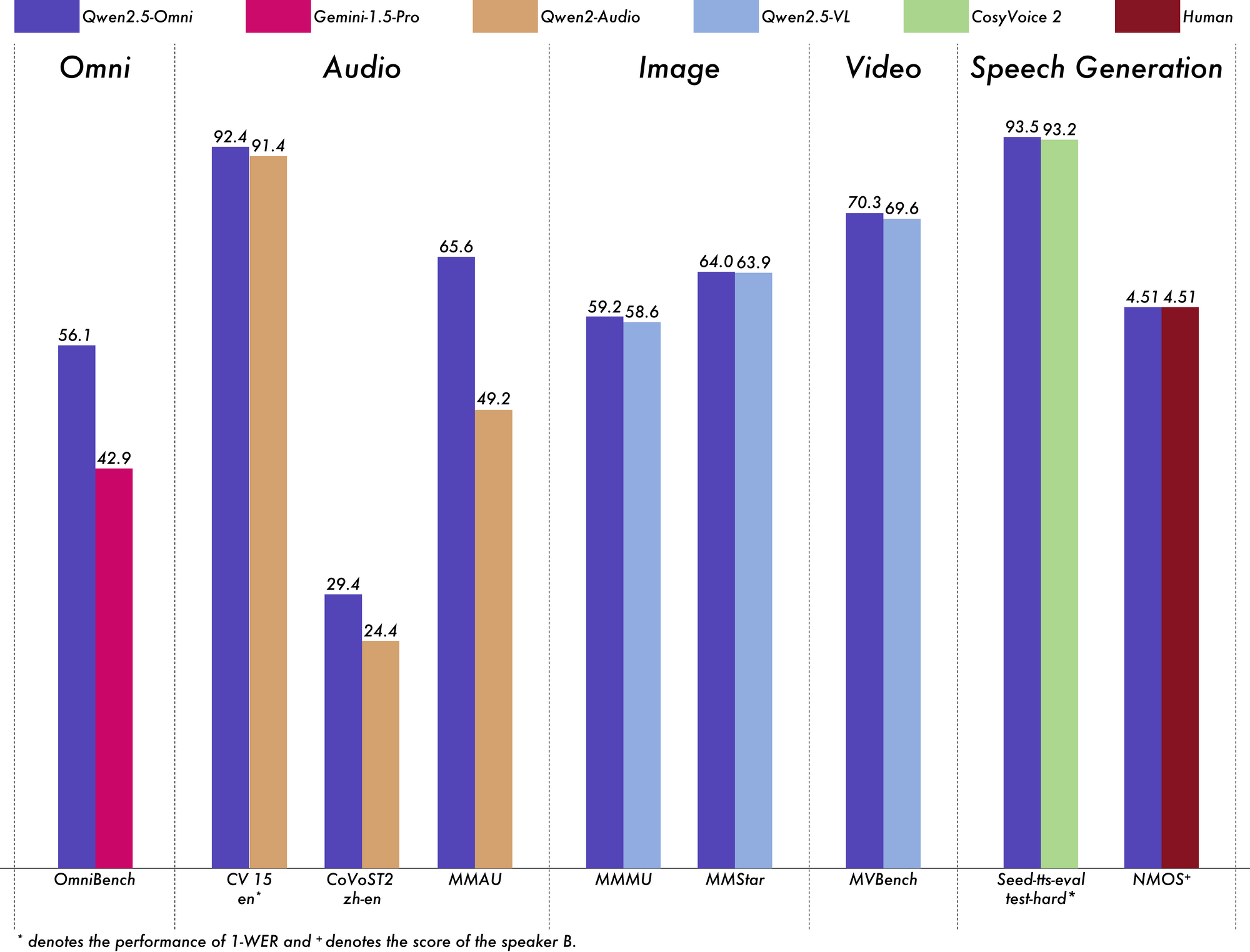
The true test of any AI model is its performance across rigorous benchmarks, and Qwen 2.5 Omni 7B delivers impressive results across the board.
Leading in Multimodal Understanding
On the OmniBench benchmark for general multimodal understanding, Qwen 2.5 Omni 7B achieves an average score of 56.13%. This significantly outperforms other models like Gemini-1.5-Pro (42.91%) and MIO-Instruct (33.80%). Its exceptional performance in specific OmniBench categories is particularly noteworthy:
- Speech tasks: 55.25%
- Sound Event tasks: 60.00%
- Music tasks: 52.83%
This comprehensive performance demonstrates the model's ability to effectively integrate and reason across multiple modalities.
Excelling in Audio Processing
For audio-to-text tasks, Qwen 2.5 Omni 7B shows near state-of-the-art results in Automatic Speech Recognition (ASR). On the Librispeech dataset, it achieves Word Error Rates (WER) ranging from 1.6% to 3.5%, comparable to specialized models like Whisper-large-v3.
In Sound Event Recognition on the Meld dataset, it achieves best-in-class performance with a score of 0.570. The model even excels in music understanding, with a 0.88 score on the GiantSteps Tempo benchmark.
Strong Image Understanding
When it comes to image-to-text tasks, Qwen 2.5 Omni 7B achieves a 59.2 score on the MMMU benchmark, remarkably close to GPT-4o-mini's 60.0. On the RefCOCO Grounding task, it reaches 90.5% accuracy, outperforming Gemini 1.5 Pro's 73.2%.
Impressive Video Comprehension
For video-to-text tasks without subtitles, the model scores 64.3 on Video-MME, nearly matching the performance of specialized video models. When subtitles are added, performance jumps to 72.4, showcasing the model's ability to integrate multiple information sources effectively.
Natural Speech Generation
Qwen 2.5 Omni 7B doesn't just understand—it speaks. For speech generation, it achieves speaker similarity scores ranging from 0.754 to 0.752, comparable to dedicated text-to-speech models like Seed-TTS_RL. This demonstrates its ability to generate natural-sounding speech that maintains the characteristics of the original speaker's voice.
Maintaining Strong Text Capabilities
Despite its multimodal focus, Qwen 2.5 Omni 7B still performs admirably on text-only tasks. It achieves strong results on mathematical reasoning (GSM8K score: 88.7%) and code generation. While there's a small trade-off compared to the text-only Qwen2.5-7B model (which scores 91.6% on GSM8K), this slight dip is a reasonable compromise for gaining such comprehensive multimodal capabilities.
Real-World Applications of Qwen 2.5 Omni 7B:
Qwen 2.5 Omni is NUTS!
— Jeff Boudier 🤗 (@jeffboudier) March 26, 2025
I can't believe a 7B model
can take text, images, audio, video as input
give text and audio as output
and work so well!
Open source Apache 2.0
Try it, link below!
You really cooked @Alibaba_Qwen ! pic.twitter.com/pn0dnwOqjY
The versatility of Qwen 2.5 Omni 7B opens up a wide range of practical applications across numerous domains.
Enhanced Communication Interfaces
Its low-latency streaming capabilities make it ideal for real-time voice and video chat applications. Imagine virtual assistants that can see, hear, and speak naturally, understanding both verbal and non-verbal communication cues while responding with natural speech.
Advanced Content Analysis
The model's ability to process and understand diverse modalities positions it as a powerful tool for comprehensive content analysis. It can extract insights from multimedia documents, automatically identifying key information from text, images, audio, and video simultaneously.
Accessible Voice Interfaces
With its strong performance in end-to-end speech instruction following, Qwen 2.5 Omni 7B enables more natural and truly hands-free interaction with technology. This could revolutionize accessibility features for users with disabilities or situations where hands-free operation is essential.
Creative Content Generation
The model's ability to generate both text and natural speech opens new possibilities for content creation. From automatically generating narration for videos to creating interactive educational materials that respond to student queries with appropriate explanations, the applications are vast.
Multimodal Customer Service
Businesses could deploy Qwen 2.5 Omni 7B to power customer service systems that can analyze customer queries from multiple channels—voice calls, video chats, written messages—and respond naturally and appropriately to each.
Practical Considerations and Limitations
While Qwen 2.5 Omni 7B represents a significant advancement in multimodal AI, there are some practical considerations to keep in mind when working with it.
Hardware Requirements
The model's comprehensive capabilities come with substantial computational demands. Processing even a relatively short 15-second video in FP32 precision requires approximately 93.56 GB of GPU memory. Even with BF16 precision, a 60-second video still needs around 60.19 GB.
These requirements may limit accessibility for users without access to high-end hardware. However, the model does support various optimizations like Flash Attention 2, which can help improve performance on compatible hardware.
Voice Type Customization
Interestingly, Qwen 2.5 Omni 7B supports multiple voice types for its audio outputs. Currently, it offers two voice options:
- Chelsie: A female voice described as "honeyed, velvety" with "gentle warmth and luminous clarity"
- Ethan: A male voice characterized as "bright, upbeat" with "infectious energy and a warm, approachable vibe"
This customization adds another dimension to the model's flexibility in real-world applications.
Technical Integration Considerations
When implementing Qwen 2.5 Omni 7B, several technical details need attention:
- The model requires specific prompting patterns for audio output
- Consistent settings for
use_audio_in_video
parameters are necessary for proper multi-round conversations - Video URL compatibility depends on specific library versions (torchvision ≥ 0.19.0 for HTTPS support)
- The model is not currently available through Hugging Face Inference API due to limitations in supporting "any-to-any" models
The Future of Multimodal AI
Qwen 2.5 Omni 7B represents more than just another AI model—it's a glimpse into the future of artificial intelligence. By bringing together multiple sensory modalities in a unified, end-to-end architecture, it moves us closer to AI systems that can perceive and interact with the world more like humans do.
The integration of TMRoPE for temporal alignment solves a fundamental challenge in multimodal processing, while the Thinker-Talker architecture provides a framework for effectively combining diverse inputs and generating appropriate outputs. Its strong performance across benchmarks demonstrates that unified multimodal models can compete with and sometimes surpass specialized single-modality models.
As computational resources become more accessible and techniques for efficient model deployment improve, we can expect to see more widespread adoption of truly multimodal AI like Qwen 2.5 Omni 7B. The applications span virtually every industry—from healthcare and education to entertainment and customer service.
Conclusion
Qwen 2.5 Omni 7B stands as a remarkable achievement in the evolution of multimodal AI. Its comprehensive "Omni" capabilities, innovative architecture, and impressive cross-modal performance establish it as a leading example of the next generation of artificial intelligence systems.
By combining the ability to see, hear, read, and speak in a single, unified model, Qwen 2.5 Omni 7B breaks down the traditional barriers between different AI capabilities. It represents a significant step toward creating AI systems that can interact with humans and understand the world in a more natural, intuitive way.
While there are practical limitations to consider, particularly regarding hardware requirements, the model's achievements point to an exciting future where AI can seamlessly process and respond to the rich, multimodal world we inhabit. As these technologies continue to evolve and become more accessible, we can expect them to transform how we interact with technology across countless applications and domains.
Qwen 2.5 Omni 7B isn't just a technological achievement—it's a glimpse into a future where the boundaries between different forms of communication begin to dissolve, creating more natural and intuitive ways for humans and AI to interact.