This guide provides step-by-step instructions for deploying Meta's Llama 4 models (Scout and Maverick) on three major platforms: AWS, Azure, and Hugging Face. These models offer advanced capabilities including multimodal processing, massive context windows, and state-of-the-art performance.
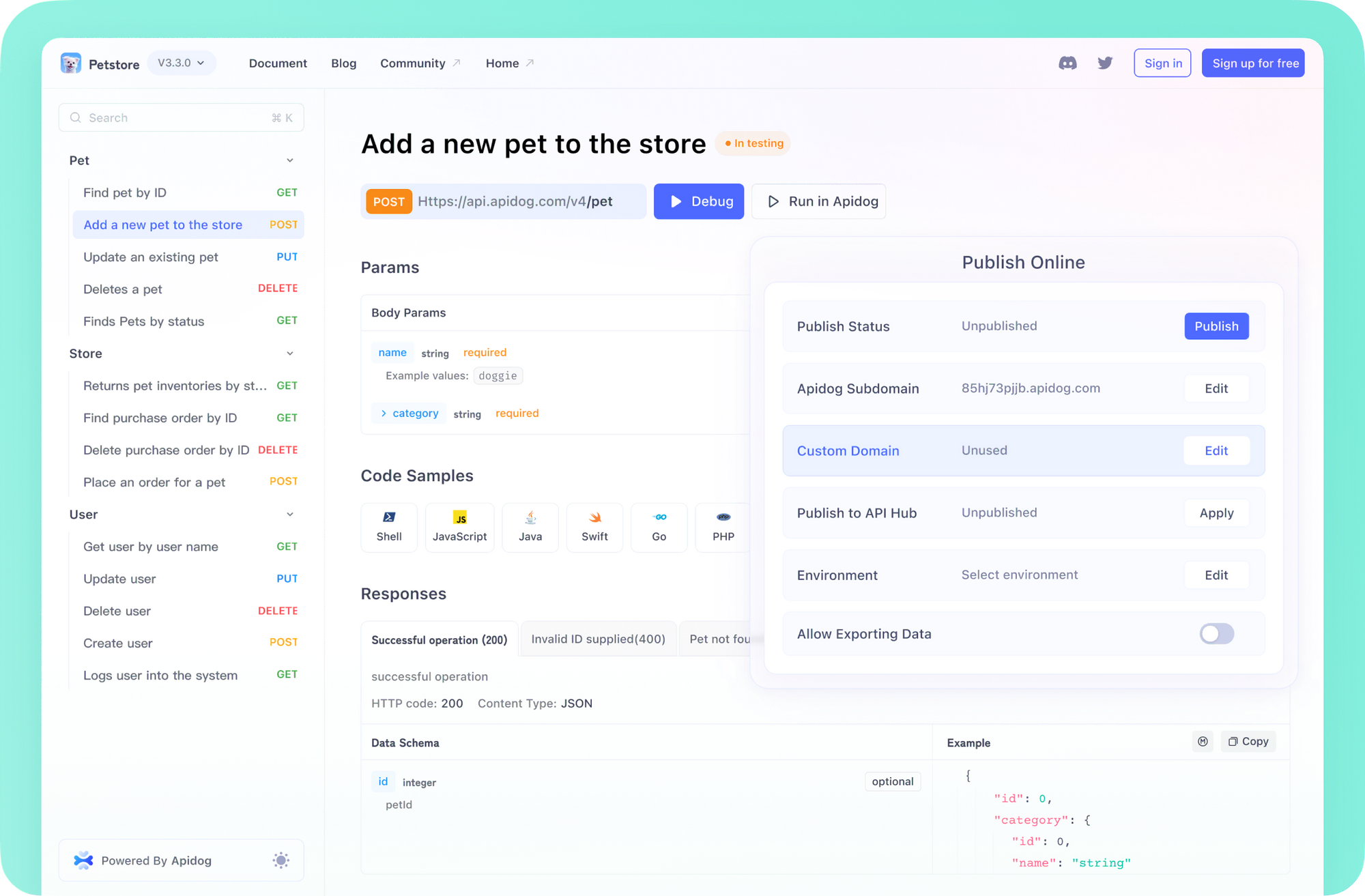
Prerequisites & Hardware Requirements for Llama 4 Deployment
- Access to Llama 4 models through Meta's license agreement
- Hugging Face account with READ access token
- AWS, Azure, or Hugging Face Pro account as needed for your deployment target
- Basic understanding of containerization and cloud services
AWS (via TensorFuse)
- Scout: 8x H100 GPUs for 1M token context
- Maverick: 8x H100 GPUs for 430K token context
- Alternative: 8x A100 GPUs (reduced context window)
Azure
(This aligns with general Azure ML guidance for large language models, but no Llama 4-specific documentation was found to confirm exact requirements.)
- Recommended: ND A100 v4-series (8 NVIDIA A100 GPUs)
- Minimum: Standard_ND40rs_v2 or higher
Hugging Face
- Recommended: A10G-Large Space hardware
- Alternative: A100-Large (premium hardware option)
- Free tier hardware is insufficient for full models
1. Deploying Llama 4 to AWS using TensorFuse
1.1 Set Up AWS and TensorFuse
Install TensorFuse CLI:
pip install tensorfuse
Configure AWS credentials:
aws configure
Initialize TensorFuse with your AWS account:
tensorkube init
1.2 Create Required Secrets
Store your Hugging Face token:
tensorkube secret create hugging-face-secret YOUR_HF_TOKEN --env default HUGGING_FACE_HUB_TOKEN=
Create API authentication token:
tensorkube secret create vllm-token vllm-key --env default VLLM_API_KEY=
1.3 Create Dockerfile for Llama 4
For Scout model:
FROM vllm/vllm-openai:v0.8.3
ENV HF_HUB_ENABLE_HF_TRANSFER=1
EXPOSE 80
ENTRYPOINT ["python3", "-m", "vllm.entrypoints.openai.api_server", \\\\
"--model", "meta-llama/Llama-4-Scout-17B-16E-Instruct", \\\\
"--dtype", "bfloat16", \\\\
"--trust-remote-code", \\\\
"--tensor-parallel-size", "8", \\\\
"--max-model-len", "1000000", \\\\
"--port", "80", \\\\
"--override-generation-config", "{\\\\"attn_temperature_tuning\\\\": true}", \\\\
"--limit-mm-per-prompt", "image=10", \\\\
"--kv-cache-dtype", "fp8", \\\\
"--api-key", "${VLLM_API_KEY}"]
For Maverick model:
FROM vllm/vllm-openai:v0.8.3
ENV HF_HUB_ENABLE_HF_TRANSFER=1
EXPOSE 80
ENTRYPOINT ["python3", "-m", "vllm.entrypoints.openai.api_server", \\\\
"--model", "meta-llama/Llama-4-Maverick-17B-128E-Instruct", \\\\
"--dtype", "bfloat16", \\\\
"--trust-remote-code", \\\\
"--tensor-parallel-size", "8", \\\\
"--max-model-len", "430000", \\\\
"--port", "80", \\\\
"--override-generation-config", "{\\\\"attn_temperature_tuning\\\\": true}", \\\\
"--limit-mm-per-prompt", "image=10", \\\\
"--kv-cache-dtype", "fp8", \\\\
"--api-key", "${VLLM_API_KEY}"]
1.4 Create Deployment Configuration
Create deployment.yaml
:
gpus: 8
gpu_type: h100
secret:
- huggingfacesecret
- vllmtoken
min-scale: 1
readiness:
httpGet:
path: /health
port: 80
1.5 Deploy to AWS
Deploy your service:
tensorkube deploy --config-file ./deployment.yaml
1.6 Access Your Deployed Service
List deployments to get your endpoint URL:
tensorkube deployment list
Test your deployment:
curl --request POST \\\\
--url YOUR_APP_URL/v1/completions \\\\
--header 'Content-Type: application/json' \\\\
--header 'Authorization: Bearer vllm-key' \\\\
--data '{
"model": "meta-llama/Llama-4-Scout-17B-16E-Instruct",
"prompt": "Earth to Llama 4. What can you do?",
"max_tokens": 1000
}'
2. Deploying Llama 4 to Azure
2.1 Set Up Azure ML Workspace
Install Azure CLI and ML extensions:
pip install azure-cli azure-ml
az login
Create Azure ML workspace:
az ml workspace create --name llama4-workspace --resource-group your-resource-group
2.2 Create Compute Cluster
az ml compute create --name llama4-cluster --type amlcompute --min-instances 0 \\\\
--max-instances 1 --size Standard_ND40rs_v2 --vnet-name your-vnet-name \\\\
--subnet your-subnet --resource-group your-resource-group --workspace-name llama4-workspace
2.3 Register Llama 4 Model in Azure ML
Create model.yml
:
$schema: <https://azuremlschemas.azureedge.net/latest/model.schema.json>
name: llama-4-scout
version: 1
path: .
properties:
model_name: "meta-llama/Llama-4-Scout-17B-16E-Instruct"
Register the model:
az ml model create --file model.yml --resource-group your-resource-group --workspace-name llama4-workspace
2.4 Create Deployment Configuration
Create deployment.yml
:
$schema: <https://azuremlschemas.azureedge.net/latest/managedOnlineDeployment.schema.json>
name: llama4-deployment
endpoint_name: llama4-endpoint
model: azureml:llama-4-scout@latest
instance_type: Standard_ND40rs_v2
instance_count: 1
environment_variables:
HUGGING_FACE_HUB_TOKEN: ${{secrets.HF_TOKEN}}
VLLM_API_KEY: ${{secrets.VLLM_KEY}}
environment:
image: vllm/vllm-openai:v0.8.3
conda_file: conda.yml
Create conda.yml
:
channels:
- conda-forge
dependencies:
- python=3.10
- pip
- pip:
- vllm==0.8.3
- transformers
- accelerate
2.5 Create Endpoint and Deploy
az ml online-endpoint create --name llama4-endpoint \\\\
--resource-group your-resource-group --workspace-name llama4-workspace
az ml online-deployment create --file deployment.yml \\\\
--resource-group your-resource-group --workspace-name llama4-workspace
2.6 Test the Deployment
az ml online-endpoint invoke --name llama4-endpoint --request-file request.json \\\\
--resource-group your-resource-group --workspace-name llama4-workspace
Where request.json
contains:
{
"model": "meta-llama/Llama-4-Scout-17B-16E-Instruct",
"prompt": "Earth to Llama 4. What can you do?",
"max_tokens": 1000
}
3. Deploying Llama 4 to Hugging Face
3.1 Set Up Hugging Face Account
- Create a Hugging Face account at https://huggingface.co/
- Accept the license agreement for Llama 4 models at https://huggingface.co/meta-llama
3.2 Deploy Using Hugging Face Spaces
Navigate to https://huggingface.co/spaces and click "Create new Space"
Configure your Space:
- Name: llama4-deployment
- License: Select appropriate license
- SDK: Choose Gradio
- Space Hardware: A10G-Large (for best performance)
- Visibility: Private or Public based on your needs
Clone the Space repository:
git clone <https://huggingface.co/spaces/YOUR_USERNAME/llama4-deployment>
cd llama4-deployment
3.3 Create Application Files
Create app.py
:
import gradio as gr
import torch
from transformers import pipeline, AutoModelForCausalLM, AutoTokenizer
import os
# Add your HF token to environment or Secrets
os.environ["HUGGING_FACE_HUB_TOKEN"] = "YOUR_HF_TOKEN"
# Load model and tokenizer with appropriate configuration
model_id = "meta-llama/Llama-4-Scout-17B-16E-Instruct"
tokenizer = AutoTokenizer.from_pretrained(model_id)
model = AutoModelForCausalLM.from_pretrained(
model_id,
torch_dtype=torch.bfloat16,
device_map="auto",
trust_remote_code=True
)
# Create pipeline
pipe = pipeline(
"text-generation",
model=model,
tokenizer=tokenizer,
max_length=2048
)
def generate_text(prompt, max_length=1000, temperature=0.7):
# Format prompt according to Llama 4 format
formatted_prompt = f"<|begin_of_text|><|user|>\\\\n{prompt}<|end_of_text|>\\\\n<|assistant|>"
outputs = pipe(
formatted_prompt,
max_length=len(tokenizer.encode(formatted_prompt)) + max_length,
temperature=temperature,
do_sample=True,
)
return outputs[0]['generated_text'].replace(formatted_prompt, "")
# Create Gradio interface
demo = gr.Interface(
fn=generate_text,
inputs=[
gr.Textbox(lines=4, placeholder="Enter your prompt here...", label="Prompt"),
gr.Slider(minimum=100, maximum=2000, value=1000, step=100, label="Max Length"),
gr.Slider(minimum=0.1, maximum=1.0, value=0.7, step=0.1, label="Temperature")
],
outputs="text",
title="Llama 4 Demo",
description="Generate text using Meta's Llama 4 model",
)
demo.launch()
Create requirements.txt
:
accelerate>=0.20.3
bitsandbytes>=0.41.1
gradio>=3.50.0
torch>=2.0.1
transformers>=4.34.0
3.4 Deploy to Hugging Face
Push to your Hugging Face Space:
git add app.py requirements.txt
git commit -m "Add Llama 4 deployment"
git push
3.5 Monitor Deployment
- Visit your Space URL: https://huggingface.co/spaces/YOUR_USERNAME/llama4-deployment
- The first build will take time as it needs to download and set up the model
- Once deployed, you'll see a Gradio interface where you can interact with the model
4. Testing and Interacting with Your Deployments
4.1 Using Python Client for API Access (AWS & Azure)
import openai
# For AWS
client = openai.OpenAI(
base_url="YOUR_AWS_URL/v1", # From tensorkube deployment list
api_key="vllm-key" # Your configured API key
)
# For Azure
client = openai.AzureOpenAI(
azure_endpoint="YOUR_AZURE_ENDPOINT",
api_key="YOUR_API_KEY",
api_version="2023-05-15"
)
# Make a text completion request
response = client.completions.create(
model="meta-llama/Llama-4-Scout-17B-16E-Instruct",
prompt="Write a short poem about artificial intelligence.",
max_tokens=200
)
print(response.choices[0].text)
# For multimodal capabilities (if supported)
import base64
# Load image as base64
with open("image.jpg", "rb") as image_file:
image_base64 = base64.b64encode(image_file.read()).decode('utf-8')
# Create chat completion with the image
response = client.chat.completions.create(
model="meta-llama/Llama-4-Scout-17B-16E-Instruct",
messages=[
{
"role": "user",
"content": [
{"type": "text", "text": "Describe this image:"},
{"type": "image_url", "image_url": {"url": f"data:image/jpeg;base64,{image_base64}"}}
]
}
],
max_tokens=300
)
print(response.choices[0].message.content)
Conclusion
You now have step-by-step instructions for deploying Llama 4 models on AWS, Azure, and Hugging Face. Each platform offers different advantages:
- AWS with TensorFuse: Full control, high scalability, best performance
- Azure: Integration with Microsoft ecosystem, managed ML services
- Hugging Face: Simplest setup, great for prototyping and demos
Choose the platform that best fits your specific requirements for cost, scale, performance, and ease of management.