The landscape of large language models (LLMs) is evolving at a breathtaking pace. Beyond simply scaling up parameter counts, leading research labs are increasingly focusing on enhancing specific capabilities like reasoning, complex problem-solving, and efficiency. Tsinghua University's Knowledge Engineering Group (KEG) and Zhipu AI (THUDM) have consistently been at the forefront of these advancements, particularly with their GLM (General Language Model) series. The introduction of GLM-4-32B-0414 and its subsequent specialized variants – GLM-Z1-32B-0414, GLM-Z1-Rumination-32B-0414, and the surprisingly potent GLM-Z1-9B-0414 – marks a significant step in this direction, showcasing a sophisticated strategy that blends foundational strength with targeted enhancements.
Want an integrated, All-in-One platform for your Developer Team to work together with maximum productivity?
Apidog delivers all your demans, and replaces Postman at a much more affordable price!
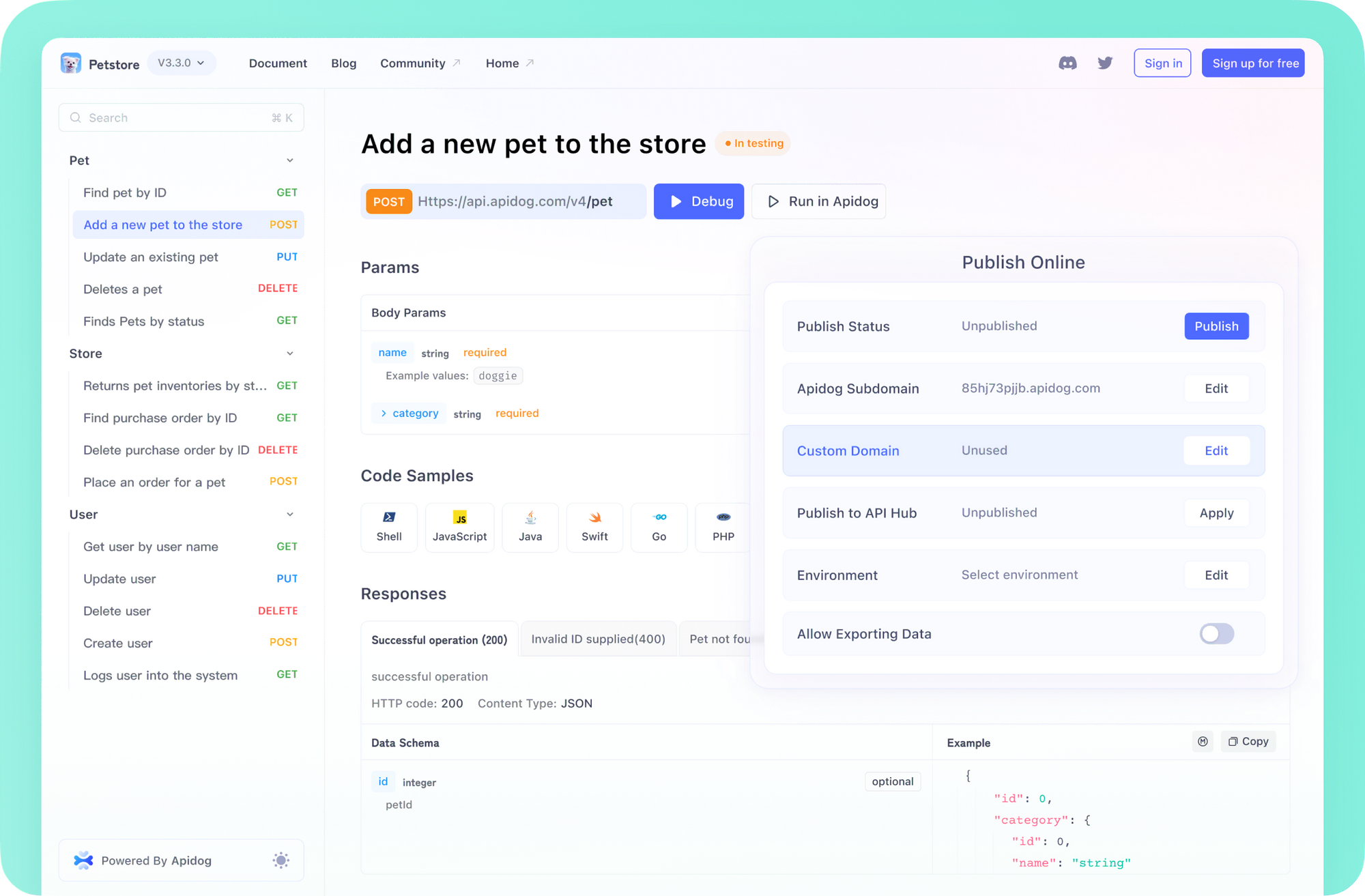
The Foundation: GLM-4-32B-0414
While the provided text focuses heavily on the derived 'Z1' models, understanding the base, GLM-4-32B-0414, is crucial. As a 32-billion parameter model, it sits in a highly competitive segment of the LLM market. Models of this size typically aim for a balance between strong performance across a wide range of tasks and manageable computational requirements compared to behemoths with hundreds of billions or trillions of parameters.
Given THUDM's history with bilingual (Chinese-English) models, GLM-4-32B likely possesses robust capabilities in both languages. Its training would involve vast datasets encompassing text and code, enabling it to perform tasks such as text generation, translation, summarization, question answering, and basic coding assistance. The '0414' suffix likely indicates a specific version or training checkpoint, suggesting ongoing development and refinement.
GLM-4-32B-0414 serves as the essential springboard for the more specialized Z1 series. It represents a powerful, generalist foundation upon which targeted capabilities can be built. Its performance on standard benchmarks would likely be strong, establishing a high baseline before specialization occurs. Think of it as the well-rounded graduate ready for advanced, specialized training.
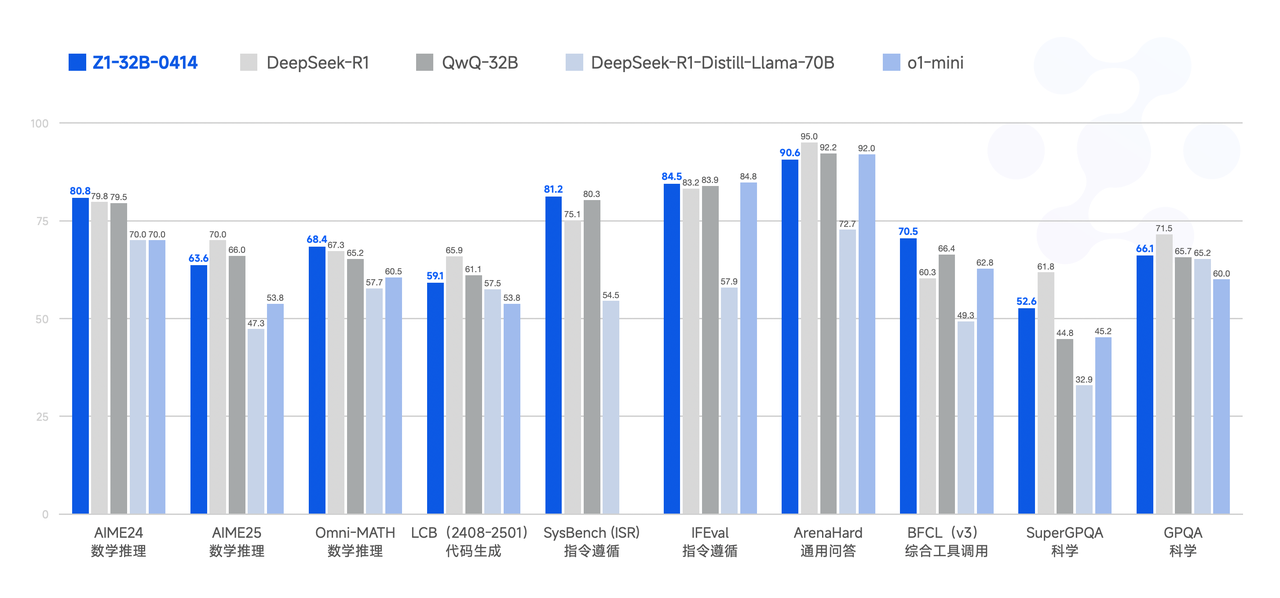
GLM-Z1-32B-0414: Sharpening the Edge of Reasoning
The first specialized variant, GLM-Z1-32B-0414, represents a focused effort to significantly boost the model's "deep thinking" and reasoning capabilities, particularly in technical domains. The development process described is multifaceted and points towards advanced fine-tuning methodologies:
- Cold Start: This intriguing term suggests initiating the specialization process not just by fine-tuning the base GLM-4-32B, but perhaps by using its weights as a smarter initialization point for a new phase of training, potentially resetting certain layers or optimizers to encourage the learning of new, distinct reasoning pathways rather than just incrementally improving existing ones.
- Extended Reinforcement Learning (RL): Standard RLHF (Reinforcement Learning from Human Feedback) aligns models with human preferences. "Extended" RL might imply longer training durations, more sophisticated reward models, or novel RL algorithms designed specifically to cultivate multi-step reasoning processes. The goal is likely to teach the model not just to provide plausible answers, but to follow logical steps, identify implicit assumptions, and handle complex instructions.
- Further Training on Specific Tasks: Explicitly targeting mathematics, code, and logic indicates that the model was fine-tuned on curated datasets rich in these areas. This could include mathematical theorems and problem sets (like GSM8K, MATH datasets), code repositories and programming problems (like HumanEval, MBPP), and logical reasoning puzzles. This direct exposure forces the model to internalize patterns and structures specific to these demanding domains.
- General RL based on Pairwise Ranking Feedback: This layer aims to ensure that the specialization doesn't come at the cost of general competence. By using pairwise comparisons (judging which of two responses is better for general prompts), the model retains its breadth of abilities while simultaneously sharpening its reasoning peaks. This prevents catastrophic forgetting and maintains overall helpfulness and coherence.
The stated outcome is a significant improvement in mathematical abilities and solving complex tasks. This suggests that GLM-Z1-32B-0414 would likely demonstrate substantially higher scores on benchmarks like:
- GSM8K: Grade School Math problems requiring multi-step arithmetic reasoning.
- MATH: Challenging competition mathematics problems.
- HumanEval / MBPP: Python code generation tasks testing functional correctness.
- Logic Benchmarks: Tests involving deductive, inductive, and abductive reasoning.
Compared to the base GLM-4-32B, one would expect Z1-32B to show marked percentage gains in these areas, potentially rivaling or even exceeding larger, more generalist models that haven't undergone such focused reasoning enhancement. It represents a strategic choice: optimizing a capable model for high-value, cognitively demanding tasks.
GLM-Z1-Rumination-32B-0414: Enabling Deeper, Search-Augmented Thought
The "Rumination" model introduces another layer of sophistication, targeting open-ended and complex problems that require more than just retrieving or reasoning over existing knowledge. The concept of "rumination" is positioned against "OpenAI's Deep Research" (perhaps referring conceptually to models capable of in-depth analysis or multi-step thought processes). Unlike standard inference where a model generates a response in a single pass, rumination implies a capacity for deeper, potentially iterative, and longer thinking.
Key aspects of GLM-Z1-Rumination-32B-0414 include:
- Deep and Long Thinking: This suggests the model might employ techniques like chain-of-thought, tree-of-thought, or internal scratchpads more extensively, or perhaps even an iterative refinement process where it critiques and improves its own intermediate outputs before producing the final response. This is crucial for tasks that lack a single, easily verifiable answer.
- Solving Open-Ended Problems: The example given – "writing a comparative analysis of AI development in two cities and their future development plans" – perfectly illustrates this. Such tasks require synthesizing information from potentially diverse sources, structuring complex arguments, making nuanced judgments, and generating coherent, long-form text.
- Scaled End-to-End RL with Graded Responses: Training involves RL, but the feedback mechanism is more sophisticated than simple preference ranking. Responses are graded based on ground truth answers (where applicable) or detailed rubrics (for qualitative tasks). This provides a much richer learning signal, teaching the model not just what is "better," but why it's better according to specific criteria (e.g., depth of analysis, coherence, factual accuracy, structure).
- Search Tool Integration during Deep Thinking: This is a critical feature. For complex, research-style tasks, the model's internal knowledge may be insufficient or outdated. By integrating search capabilities during the generation process, the model can actively seek out relevant, up-to-date information, analyze it, and incorporate it into its "rumination." This transforms the model from a static knowledge repository into a dynamic research assistant.
The expected improvements are in research-style writing and handling complex tasks requiring information synthesis and structured argumentation. Benchmarking such capabilities is notoriously difficult with standard metrics. Evaluation might rely more on:
- Human Evaluation: Assessing the quality, depth, and coherence of long-form outputs based on predefined rubrics.
- Performance on Complex QA: Datasets like StrategyQA or QuALITY that require reasoning over multiple pieces of information.
- Task-Specific Evaluations: Assessing performance on bespoke tasks similar to the comparative analysis example.
GLM-Z1-Rumination represents an ambitious push towards models that can function as genuine collaborators in complex knowledge work, capable of exploring topics, gathering information, and constructing sophisticated arguments.
GLM-Z1-9B-0414: The Lightweight Powerhouse
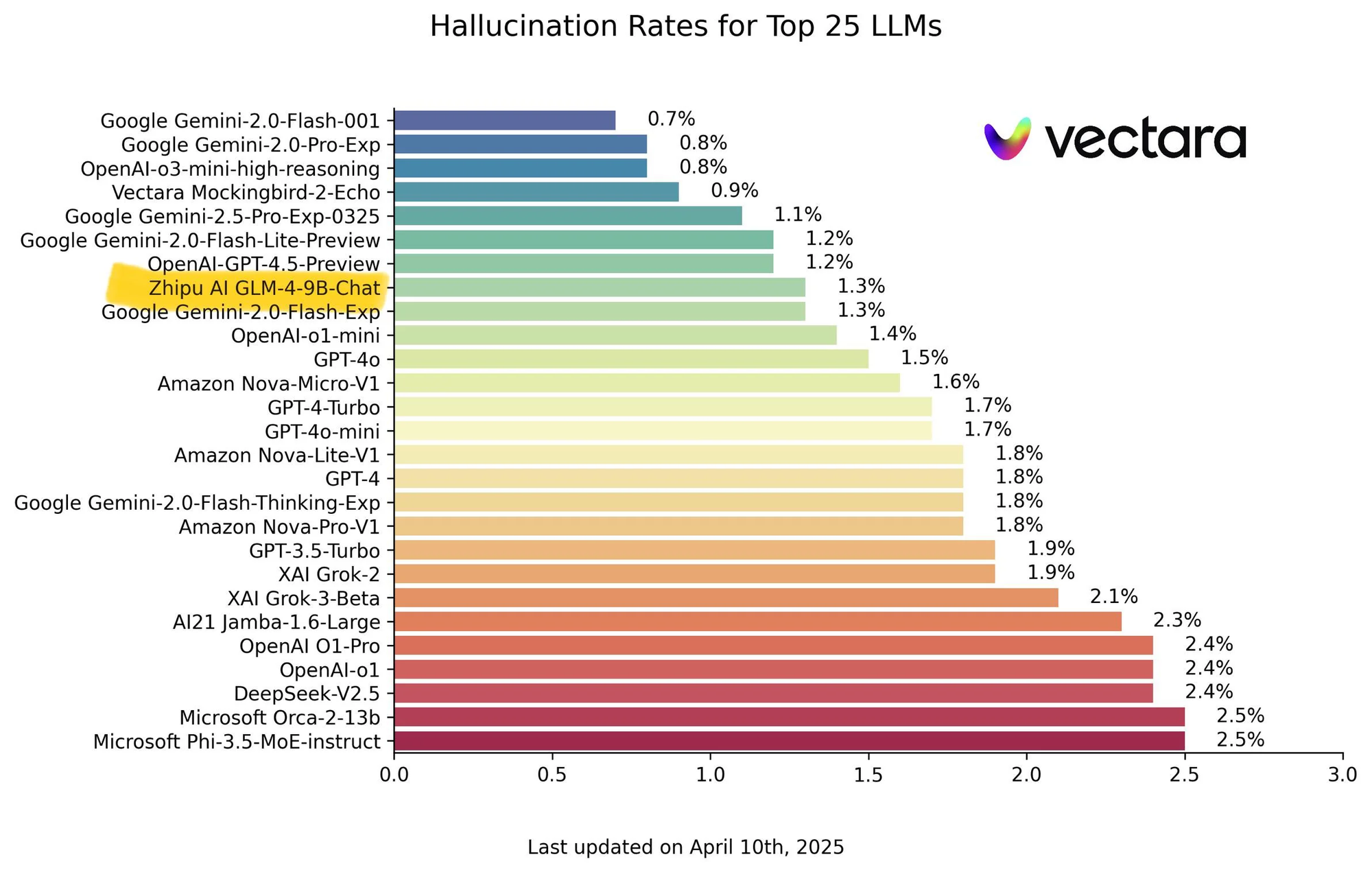
Perhaps the most intriguing release is GLM-Z1-9B-0414. While the industry often chases scale, THUDM has applied the advanced training methodologies developed for the larger Z1 models to a much smaller 9-billion parameter base. This is significant because smaller models are vastly more accessible: they require less computational power for inference, are cheaper to run, and can be deployed more easily on edge devices or standard hardware.
The key takeaway is that GLM-Z1-9B inherits the benefits of the sophisticated training pipeline: the reasoning enhancements from Z1-32B's development (likely including the task-specific training and RL techniques) and potentially aspects of the rumination training (though perhaps scaled down). The result is a model that punches significantly above its weight class.
Claims of "excellent capabilities in mathematical reasoning and general tasks" and "top-ranked among all open-source models of the same size" suggest strong performance on benchmarks relative to other models in the 7B-13B parameter range (e.g., Mistral 7B, Llama 2 7B/13B variants, Qwen 7B). We would expect GLM-Z1-9B to show particularly competitive scores on:
- MMLU: Measuring broad multi-task understanding.
- GSM8K/MATH: Demonstrating reasoning capabilities disproportionate to its size.
- HumanEval/MBPP: Showing strong coding abilities for its class.
This model addresses a critical need in the AI ecosystem: achieving an optimal balance between efficiency and effectiveness. For users and organizations operating under resource constraints (limited GPU access, budget restrictions, need for local deployment), GLM-Z1-9B offers a compelling option, providing advanced reasoning capabilities without demanding the infrastructure of much larger models. It democratizes access to sophisticated AI.
Benchmarks and Performance Analysis (Illustrative)
While specific, verified third-party benchmark numbers require ongoing tracking, based on the descriptions, we can infer the relative positioning:
Model | Parameter Size | Key Strengths | Likely High-Performing Benchmarks | Target Use Case |
---|---|---|---|---|
GLM-4-32B-0414 (Base) | 32B | Strong General Capabilities, Bilingualism | MMLU, C-Eval, General QA, Translation | Broad-purpose LLM tasks |
GLM-Z1-32B-0414 (Reasoning) | 32B | Math, Code, Logic, Complex Task Solving | GSM8K, MATH, HumanEval, Logic Puzzles | Technical problem solving, analysis |
GLM-Z1-Rumination-32B-0414 | 32B | Deep Reasoning, Open-Ended Tasks, Search Use | Long-form Eval, Complex QA, Research Tasks | Research, analysis, complex writing |
GLM-Z1-9B-0414 (Efficient) | 9B | High Performance/Size Ratio, Math/General Tasks | MMLU, GSM8K, HumanEval (relative to size) | Resource-constrained deployment |
(Note: This table structure helps visualize the differentiation. Actual scores would populate such a table in a formal report.)
The Z1-32B model would be expected to significantly outperform the base 32B model on reasoning-intensive tasks. The Rumination model might not top standard benchmarks but would excel in qualitative assessments of complex, generative tasks. The Z1-9B model's value lies in its high scores relative to other models below ~13B parameters, potentially exceeding them in math and reasoning due to its specialized training.
Deep Insights and Implications
The release of these GLM variants offers several insights into THUDM's strategy and the broader trajectory of LLM development:
- Beyond Scaling: This suite demonstrates a clear strategy of enhancing models through sophisticated training techniques rather than solely relying on increasing parameter count. Specialization is key.
- Advanced Training Methodologies: The explicit mention of cold starts, varied RL approaches (pairwise ranking, end-to-end graded), task-specific curriculum learning, and search integration highlights the complexity and innovation happening in model training beyond standard pre-training and fine-tuning.
- Reasoning as a Core Focus: Both Z1-32B variants heavily emphasize reasoning – logical, mathematical, and analytical. This reflects a growing understanding that true AI capability requires more than just pattern matching; it demands robust inferential abilities.
- The Rumination Concept: Introducing "rumination" and search-augmented thinking pushes the boundary of LLM capabilities towards more autonomous research and analysis. This could significantly impact knowledge work, content creation, and scientific discovery.
- Democratization through Efficiency: The Z1-9B model is a crucial contribution. By cascading advanced training techniques down to smaller models, THUDM makes high-performance AI more accessible, fostering wider adoption and innovation. It challenges the notion that cutting-edge capabilities are exclusive to the largest models.
- Competitive Positioning: The specific capabilities highlighted (reasoning, deep thinking, efficiency) and the implicit comparisons (e.g., "against OpenAI's Deep Research") suggest THUDM is strategically positioning its models to compete effectively in the global AI arena by focusing on distinct strengths.
Conclusion
The GLM-4-32B-0414 and its specialized Z1 offspring represent a significant advancement in the GLM family and contribute meaningfully to the AI field. GLM-4-32B provides a solid foundation, while GLM-Z1-32B sharpens the model's intellect for complex reasoning tasks in math, code, and logic. GLM-Z1-Rumination pioneers deeper, search-integrated thinking for open-ended research and analysis. Finally, GLM-Z1-9B delivers an impressive blend of advanced capabilities and efficiency, making powerful AI accessible even in resource-limited scenarios.
Together, these models showcase a sophisticated approach to AI development that values not just scale, but targeted specialization, innovative training methodologies, and practical deployment considerations. As THUDM continues to iterate and potentially open-source or provide access to these models, they offer compelling alternatives and powerful tools for researchers, developers, and users seeking advanced reasoning and problem-solving capabilities. The focus on reasoning and efficiency points towards a future where AI is not just bigger, but demonstrably smarter and more accessible.