Accessing state-of-the-art language and multimodal models often involves significant computational and financial resources. However, OpenRouter—a unified API gateway connecting users to hundreds of AI models—offers an impressive selection of free, high-quality models that deliver powerful capabilities without cost barriers. This article provides a technical exploration of the top 13 free AI models available on OpenRouter, analyzing their architectures, parameter distributions, context handling, and performance characteristics.
What is OpenRouter?
OpenRouter functions as a unified inference API for large language models (LLMs), providing standardized access to models from multiple providers through a single endpoint. It offers several technical advantages:
- API Normalization: Converts various provider-specific API formats into a standardized OpenAI-compatible interface
- Intelligent Routing: Dynamically routes requests to appropriate backends based on model availability and request parameters
- Fault Tolerance: Implements automatic fallback mechanisms to maintain service continuity
- Multi-Modal Support: Handles both text and image inputs across supported models
- Context Length Optimization: Manages token windows efficiently to maximize effective context utilization
Now, let's examine the technical specifications and capabilities of each free model available on the platform.
1. meta-llama/llama-4-maverick:free
Architecture: Mixture-of-Experts (MoE) with sparse activation Parameters: 400B total, 17B active per forward pass (128 experts) Context Length: 256,000 tokens (1 million tokens theoretical maximum) Release Date: April 5, 2025 Modalities: Text + Image → Text
Llama 4 Maverick represents Meta's advanced implementation of sparse mixture-of-experts architecture, activating only 4.25% of its total parameters during inference. This sparse activation pattern enables computational efficiency while maintaining model capacity.
Technical Specifications:
- Implements early fusion for multimodal processing with unified text-image representation
- Utilizes a routing network with top-k gating to select 2 experts per token from 128 available experts
- Employs grouped-query attention mechanisms for efficient transformer implementation
- Training corpus: ~22 trillion tokens with precision-weighted sampling
- Native multilingual support across 12 languages with efficient vocabulary encoding
- Vision encoder: 2.5B parameter specialized ViT with patch size optimization
Benchmark Performance:
- MMLU: 86.3%
- GSM8K: 92.1%
- HumanEval: 88.5%
- MMMU: 73.2%
Technical Use Cases: Multimodal reasoning, visual instruction following, cross-modal inference tasks, complex symbolic reasoning, and high-throughput API deployments.
2. https://openrouter.ai/meta-llama/llama-4-scout:free
Architecture: Mixture-of-Experts (MoE) with optimized routing Parameters: 109B total, 17B active per forward pass (16 experts) Context Length: 512,000 tokens (10 million theoretical maximum) Release Date: April 5, 2025 Modalities: Text + Image → Text
Scout represents a more deployment-optimized variant of the Llama 4 architecture, utilizing fewer experts while maintaining the same active parameter count as Maverick.
Technical Specifications:
- Reduced expert count (16 vs. 128) with optimized expert utilization
- Enhanced expert capacity with increased parameters per expert
- Employs specialized knowledge distillation techniques from Maverick
- Training corpus: ~40 trillion tokens with domain-adaptive pretraining
- Implements flash attention-2 for memory-efficient inference
- Rotation-based position embeddings for extended context handling
- Low-rank adaptation fine-tuning for instruction following
Benchmark Performance:
- MMLU: 82.7%
- GSM8K: 89.4%
- HumanEval: 84.9%
- MMMU: 68.1%
Technical Use Cases: Efficient deployments on consumer hardware, edge computing scenarios, high context-length processing with memory constraints, and multi-instance parallelization.
3. https://openrouter.ai/moonshotai/kimi-vl-a3b-thinking:free
Architecture: Lightweight MoE with specialized visual reasoning Parameters: 16B total, 2.8B active per step Context Length: 131,072 tokens Release Date: April 10, 2025 Modalities: Text + Image → Text
Kimi-VL-A3B-Thinking represents a technical achievement in efficiency-optimized multimodal modeling, delivering strong performance with minimal parameter activation.
Technical Specifications:
- Ultra-sparse MoE architecture with highly selective expert activation
- Chain-of-thought prompting integrated into pretraining objectives
- RLHF optimization with preference modeling for reasoning steps
- MoonViT encoder: Efficient visual encoder with progressive downsampling
- Implements technique-specific prompt tuning for mathematical reasoning
- Forward pass optimization for up to 60% reduced memory footprint
- 8-bit quantization support for inference optimization
Benchmark Performance:
- MathVision: 76.2% (matches performance of 7B dense models)
- MMMU: 64.8%
- MathVista: 72.3%
- VQAv2: 79.1%
Technical Use Cases: Resource-constrained visual reasoning, mathematical problem-solving with visual inputs, efficient multimodal deployment, and edge AI applications requiring visual understanding.
4. https://openrouter.ai/nvidia/llama-3.1-nemotron-nano-8b-v1:free
Architecture: Modified transformer with NVIDIA optimizations Parameters: 8B Context Length: 8,192 tokens Modalities: Text → Text
NVIDIA's contribution leverages Llama 3.1 architecture with proprietary optimizations from their Nemotron framework.
Technical Specifications:
- NeMo framework optimization for tensor parallelism
- Custom attention implementation for improved throughput
- FlashAttention-integrated computation paths
- Training with specialized data filtering and deduplication
- NVIDIA-specific multi-node distributed training optimizations
- 4-bit AWQ quantization support for deployment efficiency
- Tensor parallelism support for multi-GPU inference
Benchmark Performance:
- MMLU: 68.7%
- GSM8K: 72.9%
- HumanEval: 65.3%
- BBH: 59.8%
Technical Use Cases: NVIDIA-optimized inference environments, applications requiring efficient tensor parallelism, quantization-friendly deployments, and scenarios requiring balance between size and performance.
5. https://openrouter.ai/google/gemini-2.5-pro-exp-03-25:free
Architecture: Transformer-based architecture with recurrent memory mechanisms Parameters: Undisclosed (estimated 300B-500B) Context Length: 1,000,000 tokens Release Date: March 25, 2025 Modalities: Text + Image → Text
Gemini 2.5 Pro Experimental implements Google's latest advancements in large-scale language modeling with enhanced reasoning capabilities.
Technical Specifications:
- Implements recursive reasoning with intermediate thought step generation
- Utilizes structured recurrence for long-range dependency modeling
- Memory-efficient attention mechanisms for million-token contexts
- Multimodal fusion with hierarchical perception modeling
- Trained using Google's Pathways system for efficient model parallelism
- Incorporates Constitutional AI approaches for alignment
- State-space model components for efficient sequence modeling
Benchmark Performance:
- LMArena: #1 position (as of release date)
- MMLU: 92.1%
- GSM8K: 97.3%
- HumanEval: 94.2%
- MATH: 88.7%
Technical Use Cases: Ultra-long context processing, complex reasoning chains, scientific and mathematical task solving, code generation with complex dependencies, and multimodal understanding with extensive contextual references.
6. https://openrouter.ai/mistralai/mistral-small-3.1-24b-instruct:free
Architecture: Advanced transformer with sliding window attention Parameters: 24B Context Length: 96,000 tokens (128K theoretical maximum) Release Date: March 17, 2025 Modalities: Text + Image → Text
Mistral Small 3.1 represents Mistral AI's engineering optimization of the 24B parameter scale, delivering efficient performance with multimodal capabilities.
Technical Specifications:
- Sliding window attention mechanisms for efficient long-context processing
- Grouped-query attention implementation for memory optimization
- Vision encoder integrated with cross-attention alignment
- Byte-pair encoding with 128K vocabulary for multilingual efficiency
- SwiGLU activation functions for enhanced gradient flow
- Rotary positional embeddings for improved relative position modeling
- Function calling with JSON schema validation support
Benchmark Performance:
- MMLU: 81.2%
- GSM8K: 88.7%
- HumanEval: 79.3%
- MT-Bench: 8.6/10
Technical Use Cases: Function calling APIs, JSON-structured outputs, tool use implementations, and applications requiring balance between performance and deployment efficiency.
7. https://openrouter.ai/openrouter/optimus-alpha
Architecture: Transformer with specialized attention mechanisms Parameters: Undisclosed Modalities: Text → Text
OpenRouter's in-house Optimus Alpha model focuses on general-purpose assistant capabilities with optimizations for common API use patterns.
Technical Specifications:
- Instruction-tuned for API-oriented interactions
- Specialized token economy for efficient response generation
- Optimized for low-latency inference in API environments
- Utilizes OpenRouter's proprietary training methodology
- Implements controlled response scaling for consistent output length
Technical Use Cases: Low-latency API implementations, chatbot applications requiring consistent response characteristics, and general-purpose text generation with emphasis on instruction following.
8. https://openrouter.ai/openrouter/quasar-alpha
Architecture: Transformer with knowledge-enhanced attention Parameters: Undisclosed Modalities: Text → Text
Quasar Alpha represents OpenRouter's specialized variant focused on reasoning and knowledge representation.
Technical Specifications:
- Knowledge-enhanced attention mechanisms
- Specialized training on structured reasoning datasets
- Optimized for coherent multi-step reasoning chains
- Implements verification and self-correction mechanisms
- Trained with emphasis on factual consistency and logical reasoning
Technical Use Cases: Structured reasoning tasks, knowledge-intensive applications, fact verification systems, and applications requiring logical consistency tracking.
9. https://openrouter.ai/deepseek/deepseek-v3-base:free
Architecture: Advanced transformer with technical domain optimization Parameters: Undisclosed Modalities: Text → Text
DeepSeek V3 Base represents the foundation model from DeepSeek's latest generation, with particular strengths in technical domains.
Technical Specifications:
- Specialized pretraining with emphasis on technical corpora
- Optimized vocabulary for technical terminology representation
- Implements advanced context compression techniques
- Domain-adaptive pretraining methodology
- Technical knowledge embedding with structured representation
Technical Use Cases: Technical content generation, programming assistance requiring domain-specific knowledge, documentation generation, and technical knowledge retrieval applications.
10. https://openrouter.ai/qwen/qwen2.5-vl-3b-instruct:free
Architecture: Efficient transformer with multimodal capabilities Parameters: 3B Modalities: Text + Image → Text
Qwen2.5-VL-3B-Instruct delivers multimodal capabilities in a compact architecture optimized for efficiency.
Technical Specifications:
- Lightweight visual encoder with progressive feature extraction
- Parameter-efficient visual-language mapping
- Quantization-aware training for deployment optimization
- Memory-efficient attention implementation for multimodal fusion
- Specialized vocabulary with visual token integration
- Latency-optimized inference paths for rapid response generation
Technical Use Cases: Memory-constrained multimodal applications, edge device deployment for visual understanding, and applications requiring rapid visual processing with minimal resources.
11. https://openrouter.ai/deepseek/deepseek-chat-v3-0324:free
Architecture: Dialogue-optimized transformer Parameters: Undisclosed Modalities: Text → Text
A specialized variant of DeepSeek's base model focused on conversational interactions with enhanced dialogue management.
Technical Specifications:
- Dialogue state tracking capabilities
- Enhanced memory mechanisms for conversation history
- Turn-taking optimization for natural conversation flow
- Persona consistency through dialogue embedding techniques
- Context-aware response generation with dialogue act modeling
Technical Use Cases: Multi-turn conversational systems, dialogue systems requiring state tracking, persona-consistent chatbots, and applications with complex conversation management requirements.
12. https://openrouter.ai/deepseek/deepseek-r1-zero:free
Architecture: Reasoning-specialized transformer Parameters: Undisclosed Modalities: Text → Text
DeepSeek R1 Zero focuses on research-oriented tasks and scientific reasoning with specialized architecture modifications.
Technical Specifications:
- Enhanced multi-step reasoning with intermediate verification
- Scientific domain knowledge integration
- Specialized training on research paper corpora
- Mathematical formulation capabilities with LaTeX generation
- Technical precision optimization through specialized loss functions
Technical Use Cases: Scientific literature analysis, research assistance, technical problem solving, and applications requiring precise technical reasoning or mathematical formulations.
13. https://openrouter.ai/nousresearch/deephermes-3-llama-3-8b-preview:free
Architecture: Modified Llama 3 with specialized tuning Parameters: 8B Modalities: Text → Text
DeepHermes-3 represents Nous Research's optimization of the Llama 3 architecture for balanced performance in a compact implementation.
Technical Specifications:
- Built on Llama 3 8B foundation with specialized fine-tuning
- Instruction-tuning methodology with diverse task representation
- Implements constitutional AI principles for alignment
- DPO (Direct Preference Optimization) fine-tuning
- Enhanced reasoning abilities through synthetic data augmentation
- Optimized for versatility across multiple domains
Benchmark Performance:
- MMLU: 64.3%
- GSM8K: 67.8%
- HumanEval: 55.9%
- MT-Bench: 7.2/10
Technical Use Cases: Applications requiring balanced performance within constrained computing environments, general-purpose instruction following with resource limitations, and systems requiring efficient parameter utilization.
How to Use Openrouter API with Python
Accessing these models through OpenRouter involves a straightforward API implementation that follows OpenAI-compatible patterns. Here's a technical implementation example:
import requests
import json
API_KEY = "your_openrouter_api_key"
MODEL_ID = "meta-llama/llama-4-maverick:free" # Example model
headers = {
"Authorization": f"Bearer {API_KEY}",
"HTTP-Referer": "<https://your-app-domain.com>", # Optional for analytics
"X-Title": "Your App Name", # Optional for analytics
"Content-Type": "application/json"
}
payload = {
"model": MODEL_ID,
"messages": [
{"role": "system", "content": "You are a helpful AI assistant."},
{"role": "user", "content": "Explain quantum computing in technical terms."}
],
"temperature": 0.7,
"max_tokens": 1024,
"stream": False,
"top_p": 0.95
}
response = requests.post(
"<https://openrouter.ai/api/v1/chat/completions>",
headers=headers,
data=json.dumps(payload)
)
print(response.json())
For multimodal models, image inputs can be incorporated using base64 encoding:
import base64
# Load and encode image
with open("image.jpg", "rb") as image_file:
encoded_image = base64.b64encode(image_file.read()).decode('utf-8')
# Multimodal payload
multimodal_payload = {
"model": "moonshotai/kimi-vl-a3b-thinking:free",
"messages": [
{"role": "system", "content": "You are a helpful vision assistant."},
{"role": "user", "content": [
{"type": "text", "text": "Describe this image in detail:"},
{"type": "image_url", "image_url": {"url": f"data:image/jpeg;base64,{encoded_image}"}}
]}
],
"temperature": 0.3,
"max_tokens": 1024
}
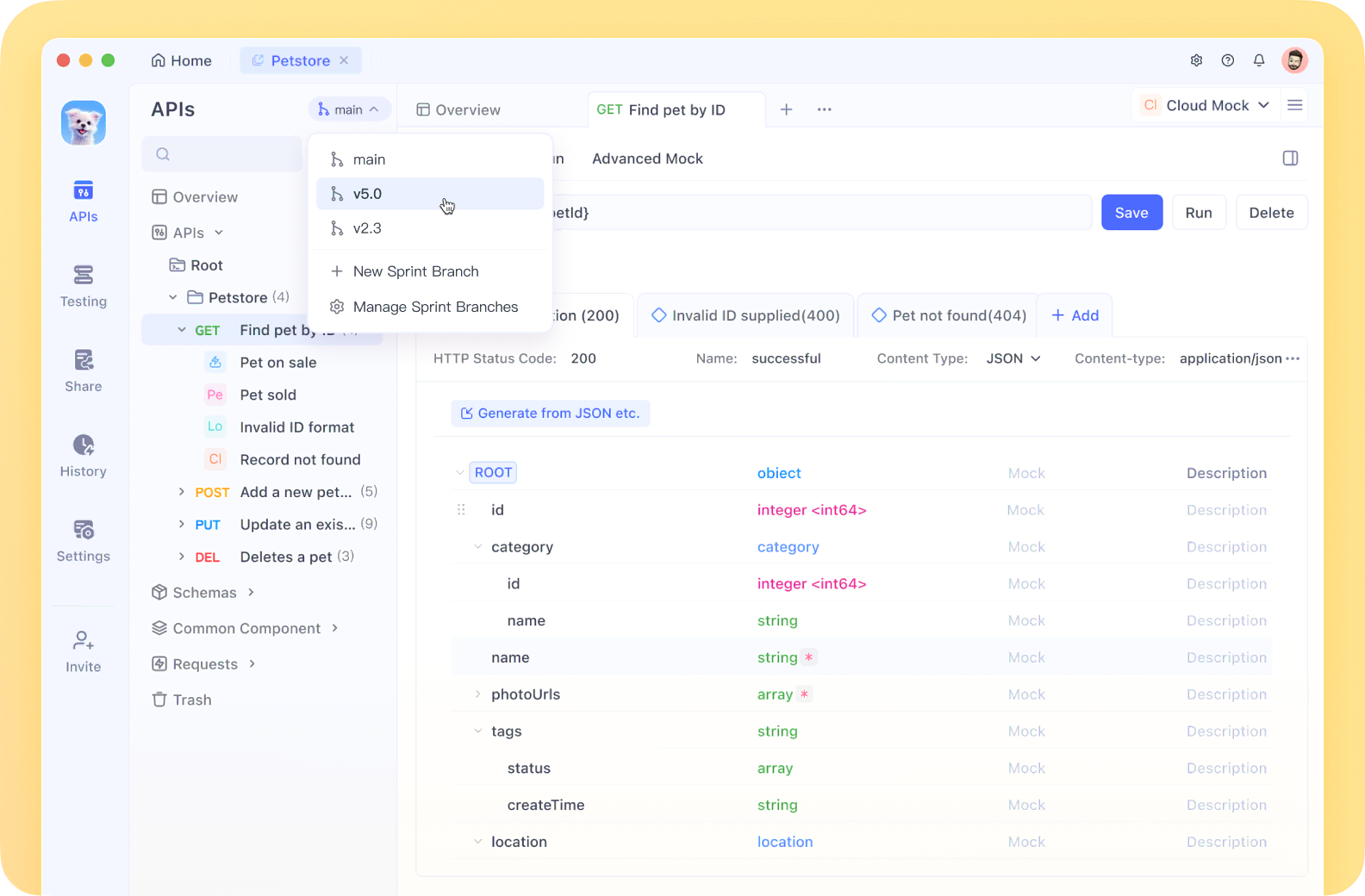
Conclusion
OpenRouter's collection of free AI models represents a significant advancement in the democratization of AI capabilities. From sophisticated MoE architectures like Llama 4 Maverick to efficient implementations like Kimi-VL-A3B-Thinking, these models offer technical capabilities that were previously accessible only through significant financial investment.
The technical diversity among these models—spanning different parameter counts, architecture approaches, multimodal capabilities, and specialized optimizations—ensures that developers can select the most appropriate model for their specific technical requirements and deployment constraints.
As the AI landscape continues its rapid evolution, platforms like OpenRouter play a crucial role in making advanced technical capabilities accessible to a broader developer community, enabling innovation without the prohibitive costs typically associated with cutting-edge AI deployment.