BigQuery MCP Server represents a significant advancement in how developers interact with their data. This innovative tool functions as an intelligent bridge between AI assistants like Claude and your BigQuery datasets, enabling natural language interactions with complex database structures. By implementing the Model Context Protocol (MCP), BigQuery MCP Server eliminates the traditional barriers between AI models and data storage systems.
At its core, BigQuery MCP Server transforms how you access and analyze data by allowing you to query your BigQuery datasets through conversational language rather than writing SQL statements manually. This capability dramatically reduces the technical expertise required to extract insights from your data and accelerates the data exploration process. Consider this typical interaction:
You: "What were our top 10 customers by revenue last quarter?"
Claude: *queries your BigQuery database and presents formatted results with analysis*
The server supports a comprehensive range of capabilities that make data interaction more intuitive:
- Natural Language Queries: Transform plain English questions into optimized SQL queries
- Resource Exploration: Access both tables and materialized views with clear type labeling
- Schema Discovery: Explore dataset structures without prior knowledge of the database design
- Safe Data Analysis: Work within configurable processing limits (1GB by default) to control costs
- Secure Access Control: Maintain data security through read-only permissions
Setting Up BigQuery MCP Server for Seamless AI Data Integration
Implementing BigQuery MCP Server requires minimal configuration while providing significant benefits for data analysis and API development. The setup process follows a straightforward path that can be completed in minutes, enabling you to quickly begin interacting with your data through natural language.
Prerequisites for BigQuery MCP Server Installation
Before beginning the installation process, ensure you have:
- Node.js 14 or higher: The runtime environment for the MCP server
- Google Cloud project: An active project with BigQuery enabled
- Authentication method: Either Google Cloud CLI installed or a service account key file
- Claude Desktop: Currently the only supported LLM interface for BigQuery MCP
Installation Options for BigQuery MCP Server
The installation process offers two approaches to accommodate different user preferences and requirements:
Option 1: Quick Installation via Smithery (Recommended)
For most users, the Smithery method provides the simplest path to implementation:
npx @smithery/cli install @ergut/mcp-bigquery-server --client claude
During this streamlined installation process, you'll be prompted for:
- Your Google Cloud project ID
- BigQuery location (defaults to us-central1)
Once configured, Smithery automatically updates your Claude Desktop configuration and restarts the application, creating a seamless setup experience.
Option 2: Manual Configuration
For users requiring more control over the installation process:
Authenticate with Google Cloud using one of these methods:
For development environments:
gcloud auth application-default login
For production environments:
# Use a service account key file with the --key-file parameter
Configure Claude Desktop by adding to your claude_desktop_config.json
:
{
"mcpServers": {
"bigquery": {
"command": "npx",
"args": [
"-y",
"@ergut/mcp-bigquery-server",
"--project-id",
"your-project-id",
"--location",
"us-central1"
]
}
}
}
When using a service account, include the --key-file
parameter pointing to your key file location.
Permissions and Security Considerations
BigQuery MCP Server requires specific permissions to function correctly while maintaining data security:
- Recommended Role:
roles/bigquery.user
- Alternative Roles: Both
roles/bigquery.dataViewer
androles/bigquery.jobUser
These permission sets ensure the server can read data and execute queries while preventing any modifications to your datasets. This read-only approach maintains data integrity while still enabling comprehensive analysis capabilities.
For production environments, consider these additional security practices:
- Use service accounts with minimal permissions
- Regularly rotate service account keys
- Monitor query usage through BigQuery audit logs
- Set appropriate query size limits to control costs
Once configured, verify your installation by asking Claude a simple question about your data, such as "What tables are available in my BigQuery project?" The system should respond with an accurate list of tables from your project, confirming successful implementation.
Enhancing API Development with Apidog MCP Server Integration
While BigQuery MCP Server focuses on database interactions, Apidog MCP Server takes a different approach by connecting your API specifications directly to AI-powered IDEs. This integration enables AI assistants to understand your API structure, accelerating development and improving code quality through context-aware assistance.
Apidog MCP Server allows developers to leverage AI assistants for generating or modifying code based on API specifications, searching through specification content, and performing various development tasks with a deep understanding of your API structure. This capability transforms how developers interact with their APIs, making development more efficient and reducing the learning curve for complex API structures.
The server works by reading and caching API specification data on your local machine, making it available to AI assistants through a standardized interface. Developers can then instruct the AI on specific tasks related to their API specifications, such as generating code for specific endpoints, updating DTOs based on schema changes, adding documentation comments, or creating MVC code structures.
Setting up Apidog MCP Server requires Node.js (version 18 or higher) and an IDE that supports MCP, such as Cursor or VS Code with the Cline plugin. The server supports three different data sources:
For Apidog project integration, you'll need to obtain an API access token and your project ID.
- The API access token can be generated from your Apidog account settings.
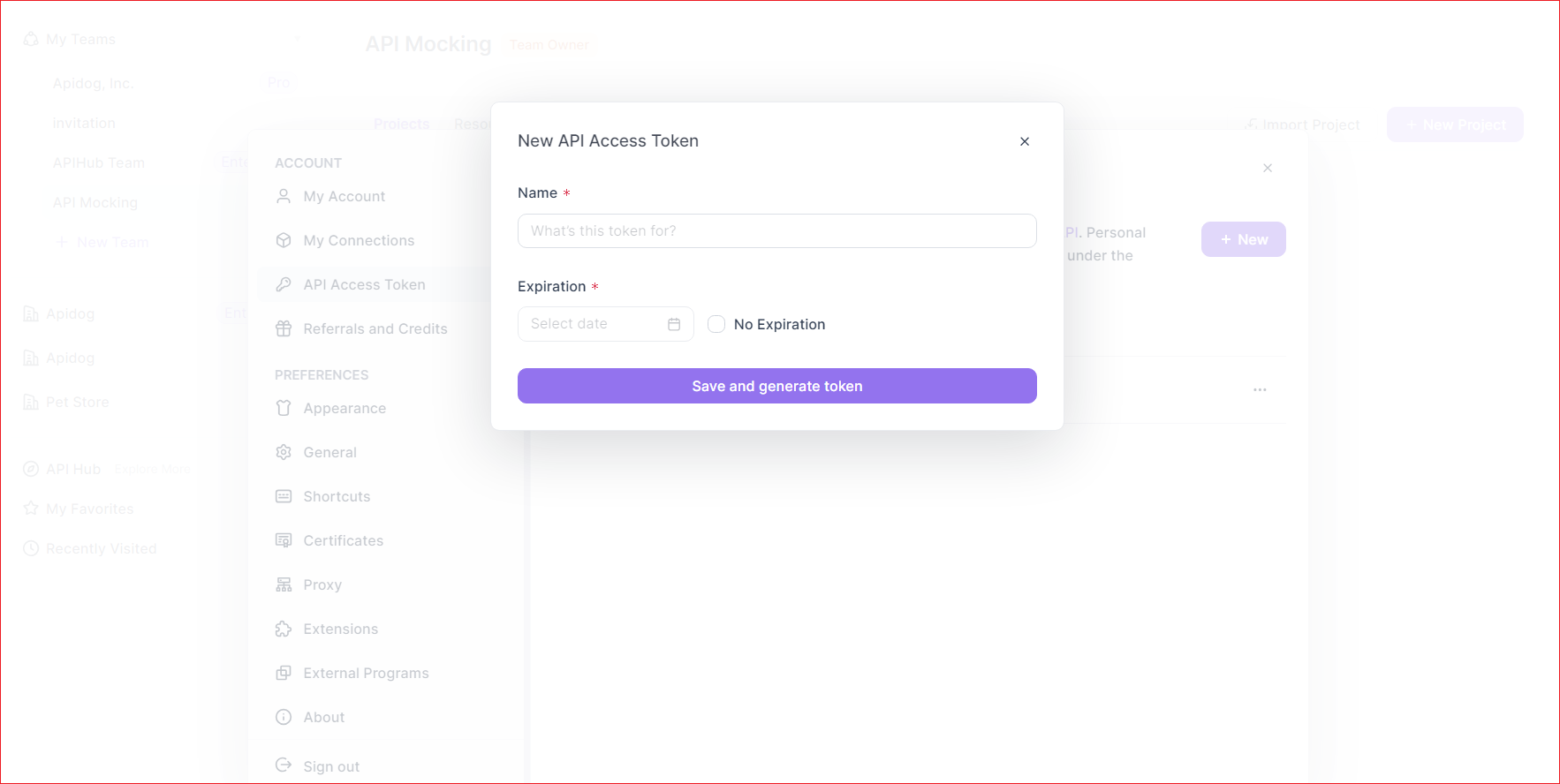
- The project ID is available in your project's basic settings.
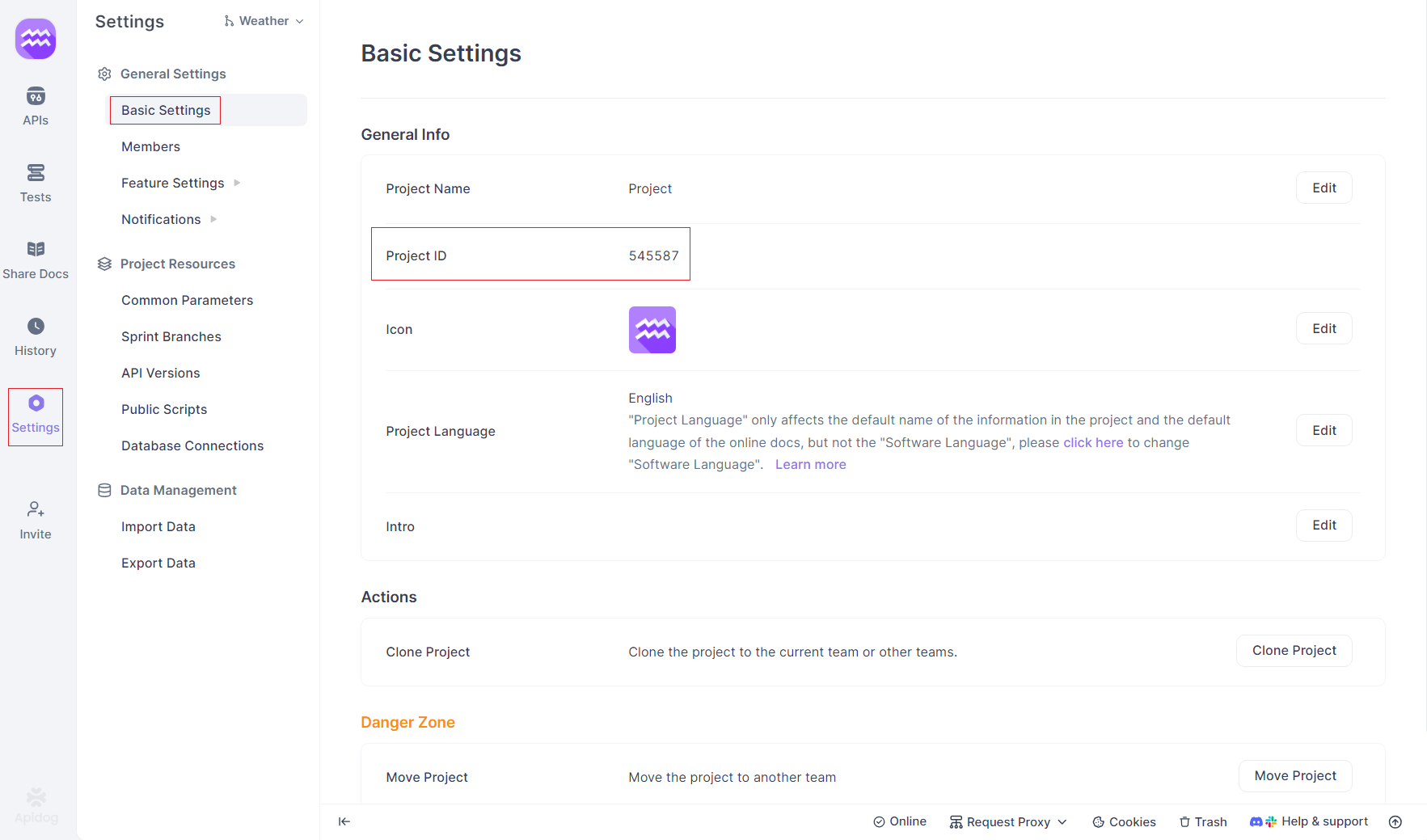
With these credentials, you can configure your MCP-compatible IDE to connect to your Apidog project.
In Cursor, for example, you would add a configuration like:
{
"mcpServers": {
"API specification": {
"command": "npx",
"args": [
"-y",
"apidog-mcp-server@latest",
"--project=<project-id>"
],
"env": {
"APIDOG_ACCESS_TOKEN": "<access-token>"
}
}
}
}
This configuration enables your AI assistant to access and understand your API specifications, allowing for more intelligent code generation and assistance.
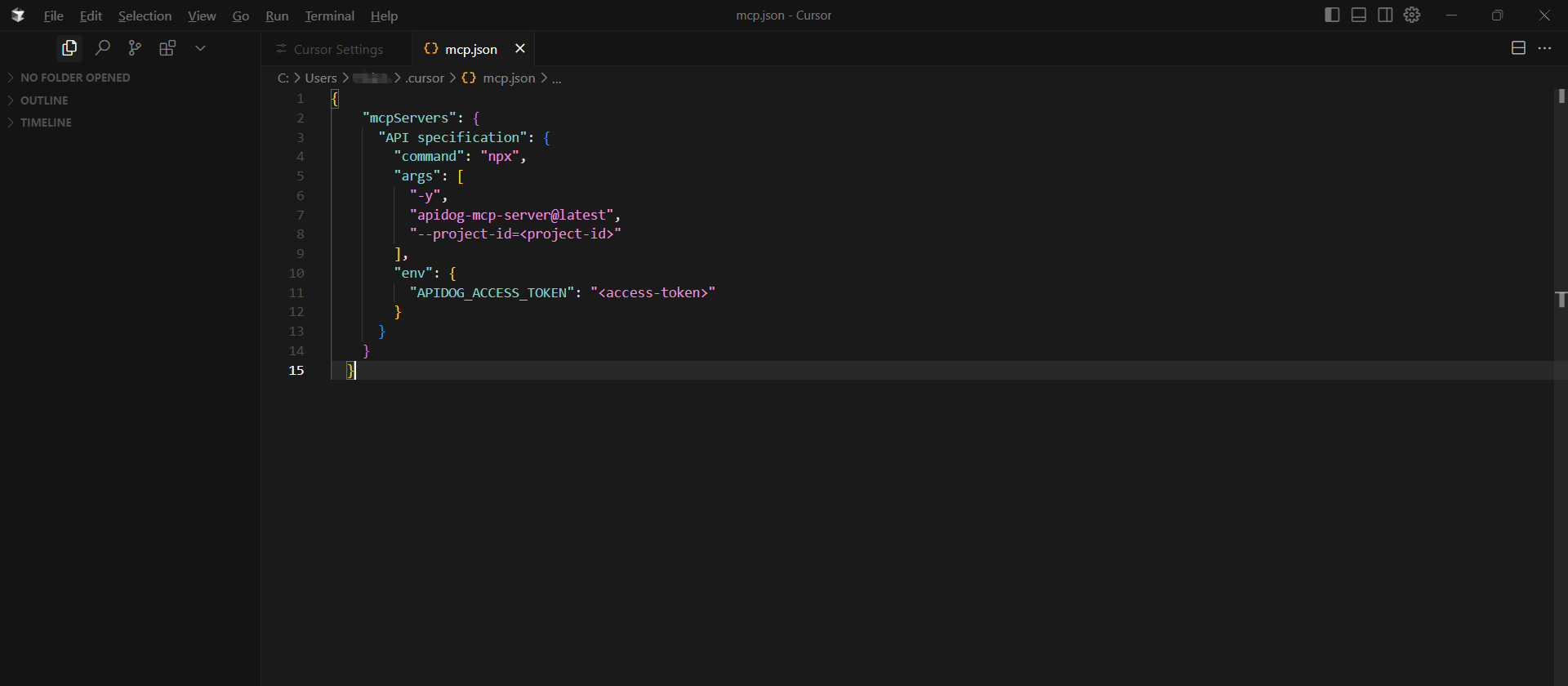
Streamlining Development Workflows with Apidog MCP for API Specifications
Apidog MCP Server significantly enhances development workflows by providing AI assistants with comprehensive knowledge of your API specifications. This integration enables developers to work more efficiently, with the AI understanding the structure, endpoints, parameters, and schemas defined in your API.
When working with Apidog MCP Server , developers can simply instruct the AI to perform tasks related to their API specifications. For example, you might ask the AI to:
- "Generate Java records for the 'Product' schema and related schemas"
- "Update the 'Product' DTO with new fields from the API specification"
- "Add comments for each field in the 'Product' class based on the API specification"
- "Generate all the MVC code related to the endpoint '/users'"
The AI assistant, with access to your API specifications through the MCP server, can then generate accurate, context-aware code that aligns perfectly with your API structure. This eliminates the need to constantly reference documentation or switch between tools when implementing API-related functionality.
Conclusion
The integration of MCP servers into development workflows represents a significant advancement in how developers interact with data and API specifications. BigQuery MCP Server enables natural language interactions with database systems, while Apidog MCP Server transforms API development by connecting specifications directly to AI assistants.
Apidog MCP Server stands out as a particularly valuable tool for API development, offering flexible configuration options for various data sources and seamless integration with AI-powered IDEs. By providing AI assistants with direct access to API specifications, the server enables more accurate code generation, improved development productivity, and enhanced code quality.