이 가이드는 메타의 Llama 4 모델(Scout 및 Maverick)을 AWS, Azure 및 Hugging Face의 세 가지 주요 플랫폼에 배포하는 단계별 지침을 제공합니다. 이 모델은 다중 모드 처리, 방대한 맥락 창 및 최첨단 성능을 포함한 고급 기능을 제공합니다.
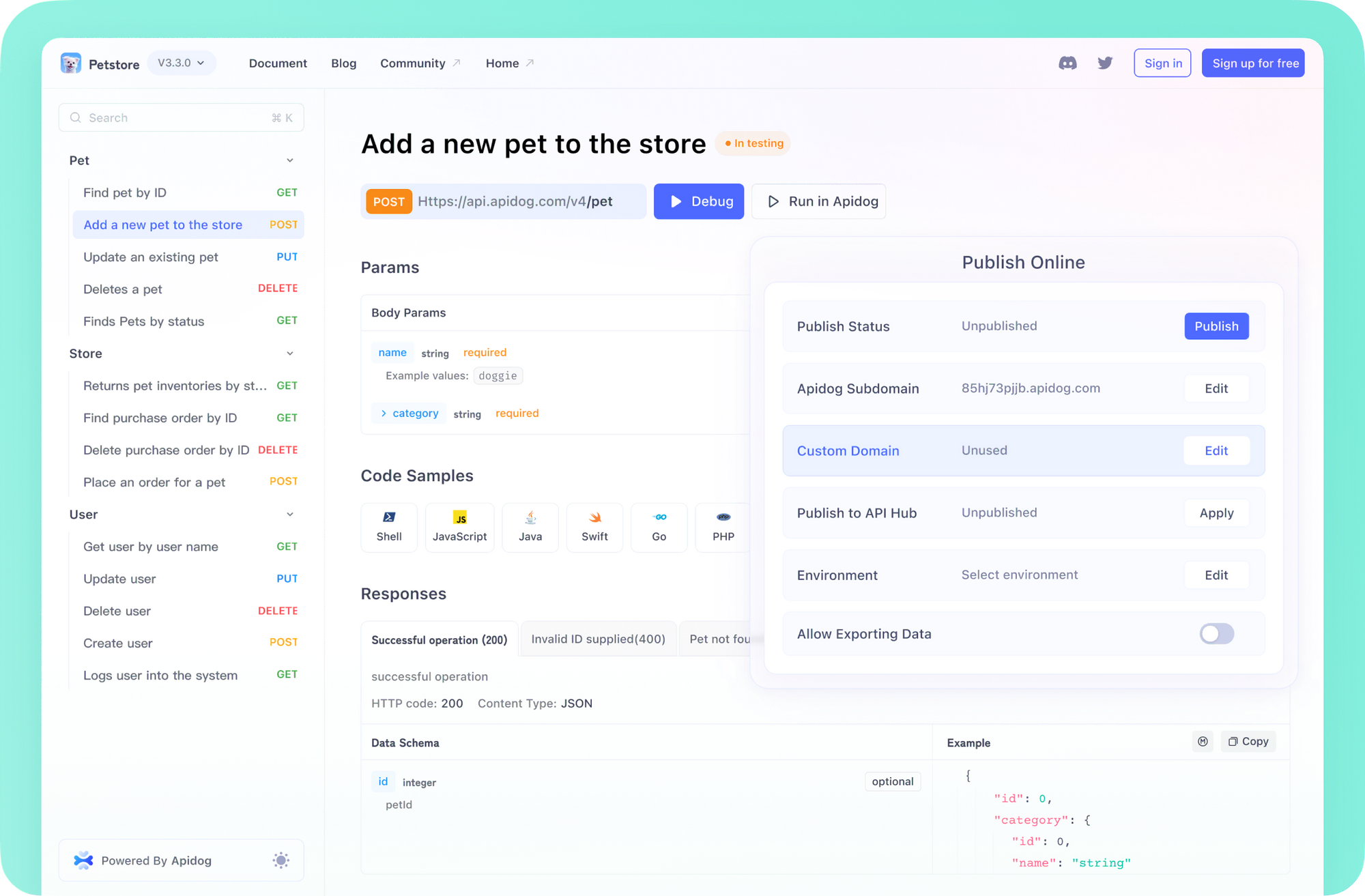
Llama 4 배포를 위한 전제조건 및 하드웨어 요구사항
- 메타의 라이선스 계약을 통한 Llama 4 모델 엑세스
- 읽기 접근 토큰이 있는 Hugging Face 계정
- 배포 대상에 필요한 AWS, Azure 또는 Hugging Face Pro 계정
- 컨테이너화 및 클라우드 서비스에 대한 기본 이해
AWS (TensorFuse를 통해)
- Scout: 1M 토큰 컨텍스트를 위한 8x H100 GPU
- Maverick: 430K 토큰 컨텍스트를 위한 8x H100 GPU
- 대안: 8x A100 GPU (축소된 컨텍스트 창)
Azure
(이는 대규모 언어 모델에 대한 일반 Azure ML 지침과 일치하지만, Llama 4에 대한 특정 문서는 정확한 요구사항을 확인할 수 없습니다.)
- 추천: ND A100 v4 시리즈 (8 NVIDIA A100 GPU)
- 최소: Standard_ND40rs_v2 또는 그 이상
Hugging Face
- 추천: A10G-Large 스페이스 하드웨어
- 대안: A100-Large (프리미엄 하드웨어 옵션)
- 무료 티어 하드웨어는 전체 모델에는 불충분합니다.
1. TensorFuse를 사용하여 AWS에 Llama 4 배포하기
1.1 AWS 및 TensorFuse 설정하기
TensorFuse CLI 설치:
pip install tensorfuse
AWS 자격 증명 구성:
aws configure
AWS 계정으로 TensorFuse 초기화:
tensorkube init
1.2 필요한 비밀 생성하기
Hugging Face 토큰 저장:
tensorkube secret create hugging-face-secret YOUR_HF_TOKEN --env default HUGGING_FACE_HUB_TOKEN=
API 인증 토큰 생성:
tensorkube secret create vllm-token vllm-key --env default VLLM_API_KEY=
1.3 Llama 4를 위한 Dockerfile 생성하기
Scout 모델을 위한:
FROM vllm/vllm-openai:v0.8.3
ENV HF_HUB_ENABLE_HF_TRANSFER=1
EXPOSE 80
ENTRYPOINT ["python3", "-m", "vllm.entrypoints.openai.api_server", \\\\
"--model", "meta-llama/Llama-4-Scout-17B-16E-Instruct", \\\\
"--dtype", "bfloat16", \\\\
"--trust-remote-code", \\\\
"--tensor-parallel-size", "8", \\\\
"--max-model-len", "1000000", \\\\
"--port", "80", \\\\
"--override-generation-config", "{\\\\"attn_temperature_tuning\\\\": true}", \\\\
"--limit-mm-per-prompt", "image=10", \\\\
"--kv-cache-dtype", "fp8", \\\\
"--api-key", "${VLLM_API_KEY}"]
Maverick 모델을 위한:
FROM vllm/vllm-openai:v0.8.3
ENV HF_HUB_ENABLE_HF_TRANSFER=1
EXPOSE 80
ENTRYPOINT ["python3", "-m", "vllm.entrypoints.openai.api_server", \\\\
"--model", "meta-llama/Llama-4-Maverick-17B-128E-Instruct", \\\\
"--dtype", "bfloat16", \\\\
"--trust-remote-code", \\\\
"--tensor-parallel-size", "8", \\\\
"--max-model-len", "430000", \\\\
"--port", "80", \\\\
"--override-generation-config", "{\\\\"attn_temperature_tuning\\\\": true}", \\\\
"--limit-mm-per-prompt", "image=10", \\\\
"--kv-cache-dtype", "fp8", \\\\
"--api-key", "${VLLM_API_KEY}"]
1.4 배포 구성 생성하기
deployment.yaml
생성하기:
gpus: 8
gpu_type: h100
secret:
- huggingfacesecret
- vllmtoken
min-scale: 1
readiness:
httpGet:
path: /health
port: 80
1.5 AWS에 배포하기
서비스 배포:
tensorkube deploy --config-file ./deployment.yaml
1.6 배포된 서비스 접근하기
배포 목록을 확인하여 엔드포인트 URL 가져오기:
tensorkube deployment list
배포 테스트:
curl --request POST \\\\
--url YOUR_APP_URL/v1/completions \\\\
--header 'Content-Type: application/json' \\\\
--header 'Authorization: Bearer vllm-key' \\\\
--data '{
"model": "meta-llama/Llama-4-Scout-17B-16E-Instruct",
"prompt": "지구에서 Llama 4. 무엇을 할 수 있습니까?",
"max_tokens": 1000
}'
2. Azure에 Llama 4 배포하기
2.1 Azure ML 작업 영역 설정하기
Azure CLI 및 ML 확장 설치:
pip install azure-cli azure-ml
az login
Azure ML 작업 영역 생성:
az ml workspace create --name llama4-workspace --resource-group your-resource-group
2.2 컴퓨팅 클러스터 생성하기
az ml compute create --name llama4-cluster --type amlcompute --min-instances 0 \\\\
--max-instances 1 --size Standard_ND40rs_v2 --vnet-name your-vnet-name \\\\
--subnet your-subnet --resource-group your-resource-group --workspace-name llama4-workspace
2.3 Azure ML에 Llama 4 모델 등록하기
model.yml
생성:
$schema: <https://azuremlschemas.azureedge.net/latest/model.schema.json>
name: llama-4-scout
version: 1
path: .
properties:
model_name: "meta-llama/Llama-4-Scout-17B-16E-Instruct"
모델 등록:
az ml model create --file model.yml --resource-group your-resource-group --workspace-name llama4-workspace
2.4 배포 구성 생성하기
deployment.yml
생성하기:
$schema: <https://azuremlschemas.azureedge.net/latest/managedOnlineDeployment.schema.json>
name: llama4-deployment
endpoint_name: llama4-endpoint
model: azureml:llama-4-scout@latest
instance_type: Standard_ND40rs_v2
instance_count: 1
environment_variables:
HUGGING_FACE_HUB_TOKEN: ${{secrets.HF_TOKEN}}
VLLM_API_KEY: ${{secrets.VLLM_KEY}}
environment:
image: vllm/vllm-openai:v0.8.3
conda_file: conda.yml
conda.yml
생성하기:
channels:
- conda-forge
dependencies:
- python=3.10
- pip
- pip:
- vllm==0.8.3
- transformers
- accelerate
2.5 엔드포인트 생성 및 배포하기
az ml online-endpoint create --name llama4-endpoint \\\\
--resource-group your-resource-group --workspace-name llama4-workspace
az ml online-deployment create --file deployment.yml \\\\
--resource-group your-resource-group --workspace-name llama4-workspace
2.6 배포 테스트하기
az ml online-endpoint invoke --name llama4-endpoint --request-file request.json \\\\
--resource-group your-resource-group --workspace-name llama4-workspace
request.json
에는 다음이 포함됩니다:
{
"model": "meta-llama/Llama-4-Scout-17B-16E-Instruct",
"prompt": "지구에서 Llama 4. 무엇을 할 수 있습니까?",
"max_tokens": 1000
}
3. Hugging Face에 Llama 4 배포하기
3.1 Hugging Face 계정 설정하기
- https://huggingface.co/에서 Hugging Face 계정 생성
- https://huggingface.co/meta-llama에서 Llama 4 모델에 대한 라이선스 계약 수락
3.2 Hugging Face 스페이스로 배포하기
https://huggingface.co/spaces로 이동하여 "새 스페이스 만들기" 클릭
스페이스 구성:
- 이름: llama4-deployment
- 라이선스: 적절한 라이선스 선택
- SDK: Gradio 선택
- 스페이스 하드웨어: A10G-Large (최고 성능을 위해)
- 가시성: 필요에 따라 비공개 또는 공개 설정
스페이스 리포지토리 복제:
git clone <https://huggingface.co/spaces/YOUR_USERNAME/llama4-deployment>
cd llama4-deployment
3.3 애플리케이션 파일 생성하기
app.py
생성:
import gradio as gr
import torch
from transformers import pipeline, AutoModelForCausalLM, AutoTokenizer
import os
# HF 토큰을 환경 변수 또는 비밀에 추가
os.environ["HUGGING_FACE_HUB_TOKEN"] = "YOUR_HF_TOKEN"
# 적절한 구성으로 모델 및 토크나이저 로드
model_id = "meta-llama/Llama-4-Scout-17B-16E-Instruct"
tokenizer = AutoTokenizer.from_pretrained(model_id)
model = AutoModelForCausalLM.from_pretrained(
model_id,
torch_dtype=torch.bfloat16,
device_map="auto",
trust_remote_code=True
)
# 파이프라인 생성
pipe = pipeline(
"text-generation",
model=model,
tokenizer=tokenizer,
max_length=2048
)
def generate_text(prompt, max_length=1000, temperature=0.7):
# Llama 4 형식에 맞게 프롬프트 포맷팅
formatted_prompt = f"<|begin_of_text|><|user|>\\\\n{prompt}<|end_of_text|>\\\\n<|assistant|>"
outputs = pipe(
formatted_prompt,
max_length=len(tokenizer.encode(formatted_prompt)) + max_length,
temperature=temperature,
do_sample=True,
)
return outputs[0]['generated_text'].replace(formatted_prompt, "")
# Gradio 인터페이스 생성
demo = gr.Interface(
fn=generate_text,
inputs=[
gr.Textbox(lines=4, placeholder="여기에 프롬프트를 입력하세요...", label="프롬프트"),
gr.Slider(minimum=100, maximum=2000, value=1000, step=100, label="최대 길이"),
gr.Slider(minimum=0.1, maximum=1.0, value=0.7, step=0.1, label="온도")
],
outputs="text",
title="Llama 4 데모",
description="메타의 Llama 4 모델을 사용하여 텍스트 생성",
)
demo.launch()
requirements.txt
생성:
accelerate>=0.20.3
bitsandbytes>=0.41.1
gradio>=3.50.0
torch>=2.0.1
transformers>=4.34.0
3.4 Hugging Face에 배포하기
Hugging Face 스페이스로 푸시:
git add app.py requirements.txt
git commit -m "Llama 4 배포 추가"
git push
3.5 배포 모니터링
- 스페이스 URL 방문: https://huggingface.co/spaces/YOUR_USERNAME/llama4-deployment
- 첫 번째 빌드는 시간이 걸리며 모델을 다운로드하고 설정해야 합니다.
- 배포가 완료되면 모델과 상호작용할 수 있는 Gradio 인터페이스가 표시됩니다.
4. 배포 테스트 및 상호작용
4.1 API 엑세스를 위한 Python 클라이언트 사용하기 (AWS & Azure)
import openai
# AWS용
client = openai.OpenAI(
base_url="YOUR_AWS_URL/v1", # tensorkube 배포 목록에서
api_key="vllm-key" # 구성된 API 키
)
# Azure용
client = openai.AzureOpenAI(
azure_endpoint="YOUR_AZURE_ENDPOINT",
api_key="YOUR_API_KEY",
api_version="2023-05-15"
)
# 텍스트 완성 요청하기
response = client.completions.create(
model="meta-llama/Llama-4-Scout-17B-16E-Instruct",
prompt="인공지능에 대한 짧은 시를 작성하세요.",
max_tokens=200
)
print(response.choices[0].text)
# 다중 모드 기능을 위한 (지원되는 경우)
import base64
# 이미지를 base64로 로드
with open("image.jpg", "rb") as image_file:
image_base64 = base64.b64encode(image_file.read()).decode('utf-8')
# 이미지를 포함한 채팅 완성 생성
response = client.chat.completions.create(
model="meta-llama/Llama-4-Scout-17B-16E-Instruct",
messages=[
{
"role": "user",
"content": [
{"type": "text", "text": "이 이미지를 설명하세요:"},
{"type": "image_url", "image_url": {"url": f"data:image/jpeg;base64,{image_base64}"}}
]
}
],
max_tokens=300
)
print(response.choices[0].message.content)
결론
이제 AWS, Azure 및 Hugging Face에서 Llama 4 모델을 배포하는 단계별 지침을 가지고 있습니다. 각 플랫폼은 서로 다른 장점을 제공합니다:
- AWS와 TensorFuse: 완전한 제어, 높은 확장성, 최상의 성능
- Azure: Microsoft 생태계와 통합, 관리형 ML 서비스
- Hugging Face: 가장 간단한 설정, 프로토타입 및 데모에 적합
비용, 규모, 성능 및 관리 용이성에 대한 특정 요구 사항에 가장 적합한 플랫폼을 선택하세요.